On Generative AI. Q&A with Bill Franks
“ One thing that I believe makes generative AI special is that the general population can directly interact with it.”
Q1. What is so special about generative AI?
One thing that I believe makes generative AI special is that the general population can directly interact with it. It isn’t limited to power users or hidden from a user beneath layers of an application. Historically, models, AI and otherwise, were behind the scenes and not something most people interacted with directly. For example, models generate recommendations for films, books, etc. However, the user simply sees these recommendations and, outside of providing feedback via ratings, doesn’t really interact with the models. Airline tickets are priced with models, but customers only see the prices generated. Generative AI applications may be some of the first where a broad range of users are intentionally interacting with the models and doing so in a sophisticated way. Also special is that the models are generating actual content as their output and not just scores, recommendations, or forecasts. Generative AI output is much more tangible and accessible to non-experts.
Q2. Why is it so popular?
I think generative AI is popular because it captures peoples’ imagination and also allows them to use their imagination. The ability to create images by simply typing in your thoughts about what you want to see is something that not only fascinates people but is also very useful when directed properly. Of course, the large language models (LLMs) and the applications that sit on top of them are taking the world by storm. Applications such as ChatGPT pull people in due to the ability to generate answers to questions quickly. I think perhaps the most useful feature of tools like ChatGPT is the way they can clean up a draft document by not just making grammar suggestions, but also proposing additional points to make or examples to include. People like being able to get a “2nd opinion” even if it is from an AI model. And, the AI model is always available to provide that 2nd opinion whereas the humans you might often go to are not.
Similarly, people have searched for images that match their needs for many years. However, stock photos tend to cover popular and common concepts. The more obscure your need, the harder it is to find an image for it. Generative AI allows you to get a bespoke image for any situation. While it can require several iterations of your prompt to get a good image, it isn’t a huge burden.
Q3. Do you use GPT-4? If yes how?
I’ll admit that I’ve not embraced LLMs in my daily life as much as some. Partially, this is due to the unclear legal and privacy protections in place. I’ve also had some underwhelming results. For example, I asked ChatGPT about myself and it got some things right and some things wrong. It even made up an award one of my books was nominated for. The reward was plausible enough that I actually went and searched to see if I had simply forgotten that the book had been nominated for the award. But, I came to the conclusion that it was just a completely fabricated hallucination. I tend to write blog length content and have gotten pretty efficient over the years. Given that I’m also usually writing about very current topics and trends, the LLMs won’t have as much information to help me. As a result, for now I am fine sticking with my usual process for writing.
However, I do make use of image generators. I am not good or efficient at generating images myself and I very much like the ability to get an AI generator to create something compelling and on point for me. Of course, I don’t always succeed. I think my failures are often because I haven’t yet figured out the best ways to word my prompts. But, I expect to keep using image generators moving forward. Once video generators evolve further, I’ll likely use those as well. Today’s video generators don’t yet produce the quality needed to be compelling, but I’m sure they soon will.
Q4. What are the legal and ethical implications of it?
There are so many! Most fundamental is the question of what data is fair to use for model training. Most of the models out there effectively scraped everything they could from the web and other accessible sources. Yet, when people or companies posted material, they didn’t anticipate that information being used to train models. I’ve discussed how these rights might be handled previously (see here). There are already lawsuits claiming that property rights were violated by generative AI model training. Google initially held back back a music generating algorithm because they feared the legal implications of artists suing over their music being used for training. They’ve now released it in a limited fashion (see here), but with some heavy constraints. It will take time to have all of these issues resolved.
It is also critical that usage policies be clarified. While there are some changes being made, a lot of generative AI applications initially declared rights to anything you provided as part of a prompt. That’s extremely problematic for companies who have intellectual property to protect. Many companies have banned most use of generative AI tools. There have been numerous stories in the media (see here) of someone from a well-known company uploading information to ChatGPT and then some of that information leaking out to other users.
One other major area for ethical consideration is what people need to do when it comes to labeling content that was AI generated. It seems fair that I should be given notice whether an image I’m seeing is real or not. It also seems fair for me to know whether an article was written by a person or by an AI process. We currently attribute content to authors as a way to help people know where the information is coming from and to gauge whether the person is a source they think they can trust. We should attribute AI generated content similarly. It gets very murky, however, when a human author uses generative AI to help create content. At what point has the AI done enough that it needs to be a co-author?
Q5. How it is being misunderstood / misused by people?
I’ve written a couple of articles about the way that people are fundamentally misunderstanding how ChatGPT is producing its answers (see here and here). In a nutshell, people are assuming that ChatGPT is “understanding” their question, matching it with underlying documents, and curating a response. That’s not how it works.
The key to what is happening is right in the name … “generative” AI. ChatGPT is literally generating a response based on probabilities. It is not matching documents or even trying to be truthful. It simply takes your prompt, reduces it down to a series of numbers, and then predicts (one by one) what the best words would be to answer your question. Due to its extensive training data, ChatGPT actually gets a lot right. It is pretty amazing how well it does, but it is literally making each answer up based on the patterns in its training data. While we call the things it gets wrong, hallucinations, in reality every answer is a hallucination. If there is a large body of information on the topic you’re asking about and that information is consistent, then ChatGPT will do a pretty good job. The more obscure your topic or the more the available training documents disagree, the more likely to get bad answers. You can see some guidance on when ChatGPT will do better and worse here.
Q6. Building Artificial General Intelligence is challenging. It remains controversial. What is your take on this?
I personally believe we’re a good way from achieving anything near general intelligence. Many people think that ChatGPT is very intelligent and approaching AGI. However, as outlined in the prior question, it isn’t really as smart as it seems. Also, it only knows what we’ve taught it explicitly. My guess is that we’ll have applications that APPEAR to be approaching AGI much sooner that we’ll have applications that actually ARE approaching AGI.
However, I also believe that we don’t have to make it to full AGI for us to have some serious problems. If an AI application is given a set of constraints, it will relentlessly pursue optimizing within those constraints. As we turn over control of key systems or infrastructure to AI models, we run the risk of the AI going down unanticipated paths due to taking our constraints too literally and to the extreme. We don’t need AGI for society to have some big problems.
Qx Anytime else you wish to add?
I’ll just clarify that the fact that I’ve raised various issues and concerns about generative AI does not mean that I am against it or that it isn’t here to stay. I think generative AI will have profound impacts, both positive and negative. My main concern is that we think things through so that we can pursue the positive while proactively minimizing the negative. It will take some time for us to figure out how to best do that. Until then, we’ll continue to see lawsuits, ethical issues, and examples of generative AI gone wrong. But that’s how it always is with new technology.
………………………………………………….
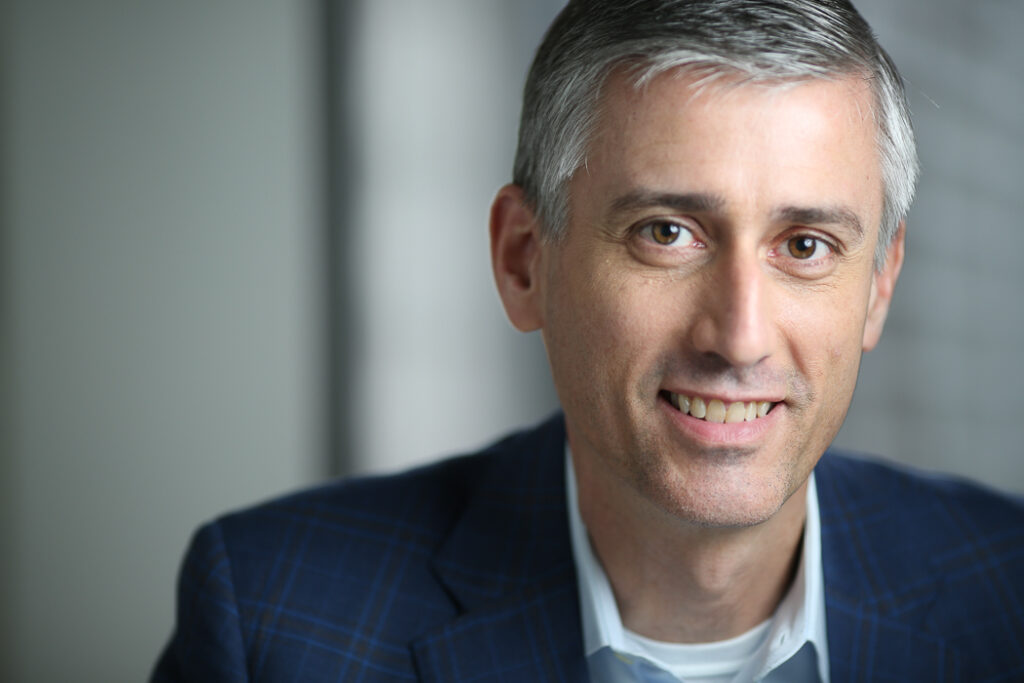
Bill Franks
Bill Franks is the Director of the Center for Data Science and Analytics at Kennesaw State University. He is also Chief Analytics Officer for The International Institute For Analytics (IIA) and serves on several corporate advisory boards. Franks is also the author of the books Winning The Room, Taming The Big Data Tidal Wave, The Analytics Revolution, and 97 Things About Ethics Everyone In Data Science Should Know. He is a sought after speaker and frequent blogger who has over the years been ranked a top global big data influencer, a top global artificial intelligence and big data influencer, a top AI influencer (both here and here), and was an inaugural inductee into the Analytics Hall of Fame. His work, including several years as Chief Analytics Officer for Teradata (NYSE: TDC), has spanned clients in a variety of industries for companies ranging in size from Fortune 100 companies to small non-profit organizations. You can learn more at http://www.bill-franks.com.