AI and Edge Computing: 7 Ways AI Is Bound to Change the Future
Edge computing is a distributed information technology architecture in which client data is processed at the periphery of the network, as close to the originating source as possible. The move toward edge computing is driven by mobile computing, the decreasing cost of computer components and the sheer number of networked devices in the IoT world. Depending on the implementation, time-sensitive data in an edge computing architecture may be processed at the point of origin by an intelligent device or sent to an intermediary server located in close geographical proximity to the client. Data that is less time sensitive is sent to the cloud for historical analysis, big data analytics and long-term storage.
The smart devices have become even more intelligent and self-contained with artificial intelligence (AI). Since AI is growing rapidly at the edge of the network, we decided to put together a list you can easily skim through and see how it is changing the future of edge computing.
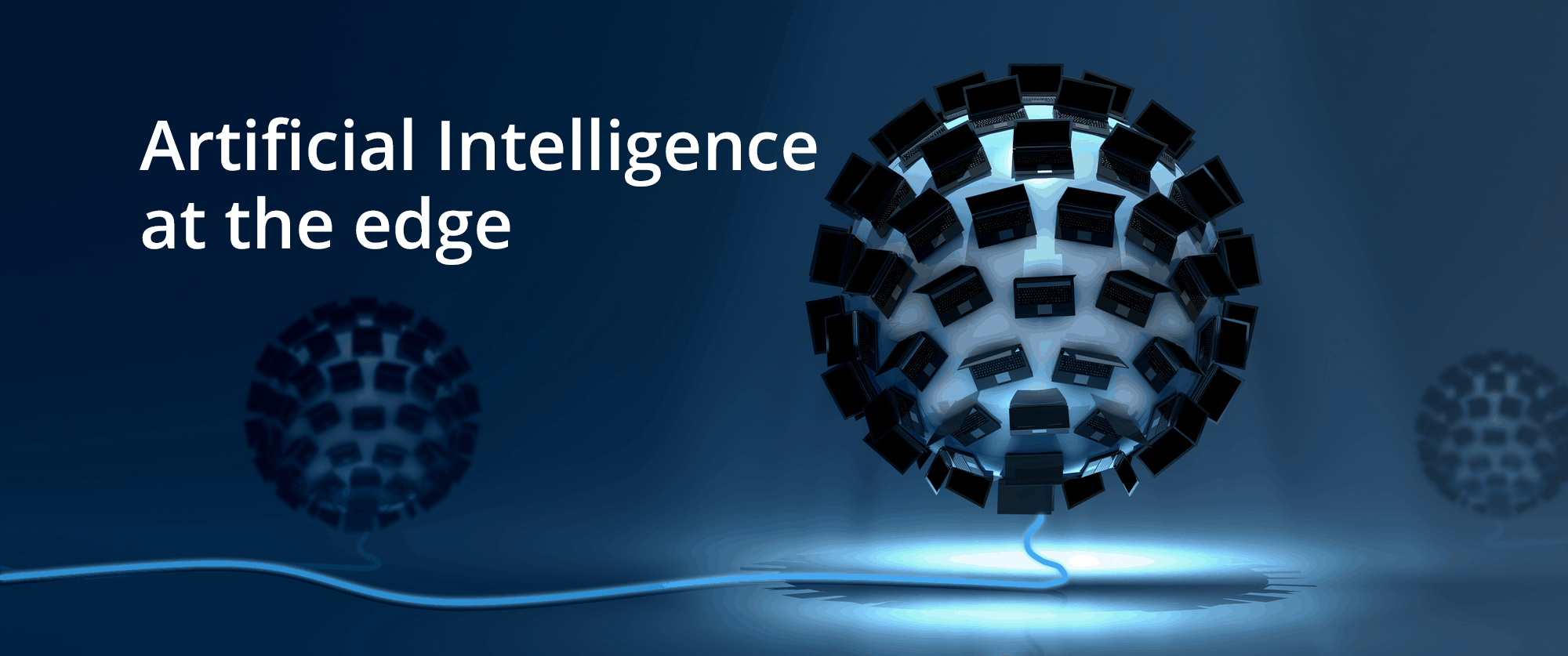
Cut-Down Expenses and Improved System Efficiency
In a cloud-based environment, all data goes to the cloud where it is processed. Thanks to edge computing, only a small portion of data ends up on the cloud data centers, while the big chunk of it remains distributed across the nodes in the network residing in edge databases.
When processing the data, the software tools use the resources of the edge computing network. With machine learning and AI, these processes can be optimized to lower the load on cloud data centers.
In most cases, it is much more efficient to process data near its source and send only the data that has value over the network to a remote data center. Instead of continually broadcasting data about the oil level in a car´s engine, for example, an automotive sensor might simply send a summary of data to a remote server periodically. Or, a smart thermostat might only transmit data if the temperature rises or falls outside acceptable limits. Or, an intelligent Wi- Fi security camera aimed at an elevator door might use edge analytics and only transmit data when a certain percentage of pixels significantly changes between two consecutive images, indicating motion.
Edge computing can also benefit remote office/branch office environments and organizations that have a geographically dispersed user base. In such a scenario, intermediary microdata centers or high-performance Raima servers can be installed at remote locations to replicate cloud services locally, improving performance and the ability for a device to act upon perishable data in fractions of a second.
Over time AI will be able to delegate the load needed for data processing efficiently, which will free up the cloud data centers and make them available for other tasks.
By doing so, AI will reduce the costs associated with the constant need to transfer data from devices to the cloud and vice versa and improve system efficiency overall.
Advanced-Data Processing and the Importance of Data Management
The more devices you have in the edge computing environment, the more data you will have to deal with. Unstructured, inaccurate, and inconsistent data standards can render all that computing power useless and deprive you of actionable insights.
Since AI is there to automate things and speed up the data processing workflow, companies have to ensure that data is ready for advanced data processing 24/7.
Hence the importance of data management. The data edge center is not in one place. It is a network of devices. A DBMS like Raima Database Manager is made for device level applications out on the edge running on odd hardware and software combinations with limited resources. AI accesses the database to analyze the data and generate reports.
When data is spread across thousands of sites and nodes, it becomes too complex even for AI to efficiently analyze it. Innovations in edge databases and data management practices enable operators and developers to have a holistic view of the data, even if it is spread all over the place.
With good data management practices and a solid edge database in place, AI will make the edge computing environments more reliable and efficient. For instance, AI will be able to allocate resources automatically to meet the required response times and return the data to apps and devices whenever it’s needed.
Improved Operational Reliability
Although quite reliable, edge computing still has some flaws, which greatly affect edge computing network reliability. The first challenge is maintaining optimal network bandwidth.
When there is a lot of data on the network, and companies want to analyze it, the request will result in a network bandwidth shift. Some of the nodes may end up being cut out, resulting in inaccurate data analysis.
Then there is the matter of distributed computing. The network has to function as a big brain for the entire operation to be reliable. Unequal computing distribution means more processing time, which translates into increased costs. By using an edge database that stores the data locally on each device you can ensure data integrity even when the network is unstable.
AI is bound to resolve all these pain points. By learning the patterns and having access to the processing power of every node in the edge computing network, AI can automatically optimize the processes. Combine this with an edge database to handle the data efficiently and reliably to achieve optimal bandwidth usage, optimized computing distribution, and no latency in the system.
Over time AI is capable of learning the intricate design of edge computing systems, data analysis patterns, and predict bandwidth and processing requirements to seamlessly dedicate resources and keep the edge computing network operational at all times.
Real-time Predictions Made Possible
Imagine having the ability to leverage all data on the edge computing network to instantly generate an insightful report. These types of requests take time if you do not pre-process the data in an edge database. Pulling the data from nodes up to a cloud system to process it stretches out the edge computing network’s resources and takes time.
Thanks to AI and local database management systems, edge computing networks will be able to perform advanced data processing to deliver real-time predictions based on the parameters you put in.
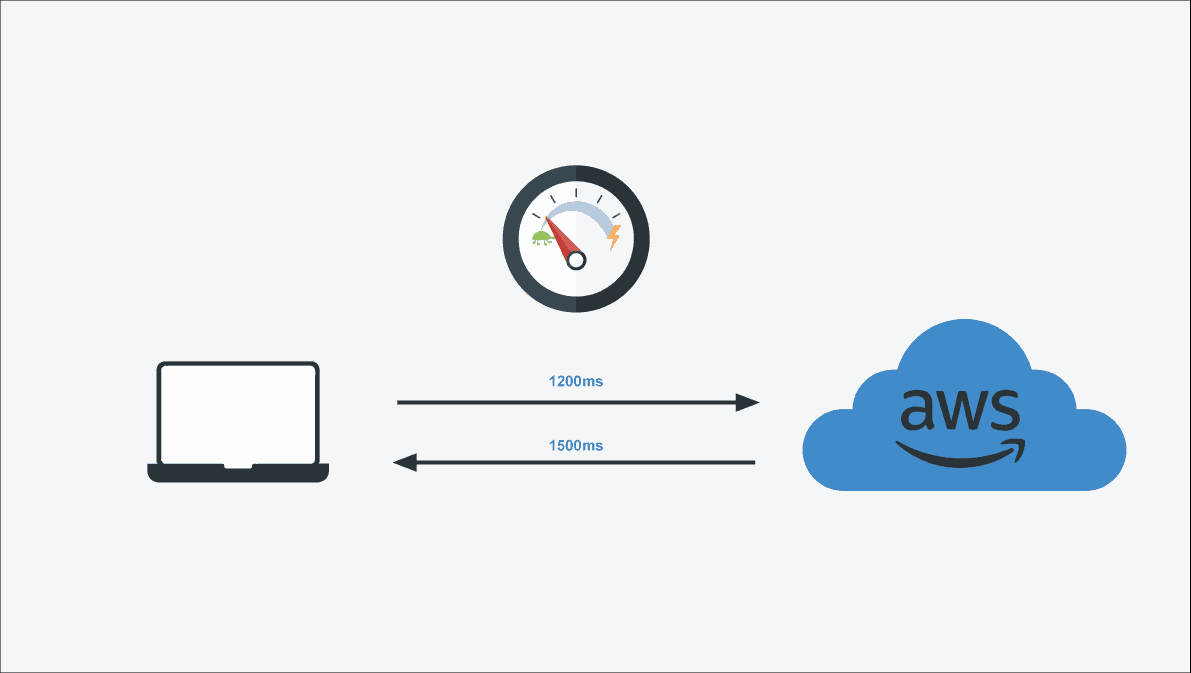
Ensure Offline-capability, Reliability and Security
Most applications will at some point lose their internet connection and many use cases require offline capability – for example, autonomous vehicles, critical applications e.g. Edge databases enables applications to always work – no matter if the device is connected to the internet or not, providing a seamless user experience. At the same time, Edge databases like RDM ensures that data is reliably stored and never lost with ACID compliant transactions, the gold-standard in data management.
AI has found its role in data security and, and it will help organizations deal with the edge computing security challenges. With the ability to identify weaknesses and vulnerabilities in the network, and immediately alert when one is being exploited, AI will make edge computing networks more secure. Combine AI with a fast and secure database system to keep your data safe at all times.
5G, Edge Databases and Quantum Computing in Line to Increase AI Efficiency
5G and quantum computing technologies are one step away from coming to our lives. 5G is already available in some parts of the world and the edge databases become more vital.
We know that 5g increases network coverage and responsiveness while lowering latency. This will result in an immense amount of data on the edge and to store and process that data securely in a fast edge database will be critical.
5G, edge databases and quantum computing will enable AI to be even more efficient in the edge computing environments in terms of delegating tasks, optimizing bandwidth, delivering real-time predictions, and boosting the system’s security.
AI will, without any doubt, play a pivotal role in edge computing networks. The complexity of edge computing grows with the number of nodes and the amount of data.
Thanks edge databases and AI’s ability to learn and adapt, we will see increased operational reliability of edge computing networks, advanced data processing in real-time, and edge computing more secure. Learn more about Raima’s edge database and download a free trial.
Share Share on Twitter
Sponsored by Raima.