On Data and FAIR principles. Q&A with Kees van Bochove
Q1. FAIR stands for Findability, Accessibility, Interoperability and Reusability. For many organisations, where large data assets have been generated and acquired over a long period of time, it is challenging to establish and maintain these FAIR Data principles. What is your take on this?
It’s true that adopting a FAIR Data strategy can be quite hard, especially for large companies. In my experience this has nearly always to do with company culture: the established processes often need to be changed to be compatible with FAIR Data. If you take a large pharma company for example, it’s of course very data and knowledge intensive in all departments from early research to marketing. But there are also a lot of data silo’s and disparate information systems, for understandable and in principle good reasons. Data and information are key assets for the departments that generate and manage them, and keeping control over the data assets allows you to control the associated business processes as well. Viewing data as company assets that should as much as possible be findable, accessible and interoperable for both humans and machines to re-use is nothing short of revolutionary from that perspective. We call this GO-CHANGE in GO-FAIR speak. It is much easier for companies that have been built with this mindset from the start.
And, to be fair, it’s much the same for science in general: you would be amazed how many scientists even today prefer to keep their scientific data to themselves rather than making it FAIR, even though this would greatly benefit the open science movement and could speed up the progress in the scientific field considerably. For example, the life sciences field is still lagging behind astronomy with regards to FAIR data for all kinds of reasons. Luckily many steps are now taken to improve this by research funders such as NIH, governments including the European Commission, building on groundwork by the RDA, DTL, ELIXIR, GO-FAIR etc.
Q2. What are the criteria you use to select the data to be integrated?
Data interoperability is a fascinating topic. I’m a software engineer by training myself, but I have to say, people are way too addicted to software. The problem with software is that is typically only lasts or at least should last for a few years, and often even shorter than that, but it often writes data like no other system will ever have to read it. We call that non-interoperable data. Quality data however has a much longer useful lifespan. What we often encounter when we make data FAIR with The Hyve, is that the data is trapped in all kind of software systems, and much of our work becomes explicating the context and meaning of software-generated data. Or even worse, doing that with spreadsheets compiled by humans because the software they use are not properly aligned with the business processes they are supposed to support. The same goes for data from lab instruments which too often is still in some proprietary format.
The FAIR principles say that all (meta)data should be stored using a formal knowledge representation which is shared and broadly applicable, a really high bar to meet. So we typically start by looking at the main pain points in the current organizations, build ‘use cases’ and then prioritize the data that could support these use cases. Often these have to do with even finding relevant datasets, especially in large organizations. Again, you would be amazed once you start looking at it from a pure data asset angle, how many business critical pieces of data are not readily available even for major business stakeholders, let alone for decision support algorithms.
Q3. What are the most common problems you encounter when integrating data from different sources and how you solve them?
The first FAIR principle is that every dataset should have a globally unique, persistent identifier. It’s very fitting that it is the first one, because without this you cannot realize any sensible data organization strategy. Yet, so often current information systems do not assign identifiers to datasets at all, or if they do, they are often not opaque, not globally unique, and also not properly registered elsewhere. So resolving id’s, tracking down codes, and assigning PIDs can be a major chunck of work and is sometimes really problematic. Another hard problem is context. The FAIR principles are aspirational, meaning that they are a great idea but also really hard if not impossible to fully realize. Principle R1 is a great example of this: making the context of a dataset completely explicit is typically impossible, you have to find a pragmatic balance.
Q3. In particular, how do you manage semantic data integration, curation processes supporting data quality and annotation, and appropriate application of data standards?
The trick, as explained earlier, is to look at data independent from the information systems it is attached to, but instead see in the context of the larger organization. To do that, it often helps to build a semantic model that is primarily informed by the organization’s business processes and industry standards, which you can then use as a sort of Rosetta stone to specify the dataset context as well as to make data interoperable and link them syntactically and semantically.
Q4. How do you judge the quality of data, before and after you have done data integration?
Quality of data is subjective, because it depends on what you intend to use the data for. The same series of images could be perfectly fine for training an image-recognition algorithm, but useless to train a clinical decision making algorithm. A more objective way of describing the data context is specifying the data lineage or provenance, how it came to be, and let the users decide on whether the data is fit for their purposes based on that. However, of course there are often automated checks you can do as well, for example on data consistency across sources, and general intelligence such as the rules of physics. For example, a dataset that has medical data should not have subjects with a negative age, or pregnant males.
Q5. How do you mitigate the risk that data integration introduces unwanted noise or bias?
Interesting question. You could argue that data doesn’t have any noise or bias, a data element is called a ‘datum’ or given after all. Noise, bias etc. are introduced by either the way the data was captured, or by our usage of the data, and are only meaningful in a certain context. So when you perform data integration, you have to be mindful that you accurately capture the provenance of the source data with the new integrated dataset. Doing so allows the data user to assess the usefulness of the data for their purpose. In the end it all depends again on the intended use. For example, in the OMOP data model, we map medical history data to a common model and vocabulary, which often loses aspects of the original data. However, you gain the ability to do large-scale analysis in multiple databases in a systematic and reproducible manner. And by also storing the source codes, you can always dive deeper and query on original terms as well, or decide to look at the original data.
Q6. How do mitigate the risk that the AI-algorithms produce Allocative harm and or Representation harm (*)
I’m not an expert in AI, my focus is more on the optimal data organization to enable AI. But it’s clear that this is an important topic. In The Netherlands, the country where I live, the first article of the Constitution establishes discrimination as unlawful. (“All persons in the Netherlands shall be treated equally in equal circumstances”.) So this is clearly something that any organization leveraging AI needs to take into account. Algorithms are not above the law.
Q7. How do you manage advanced analytics with the FAIR principles?
The FAIR principles are about maximizing the reusability of data for humans and machines alike, but purposely do not specify how the data should be used, that’s not in scope. Advanced analytics is just one of the many applications you can build on top of well-structured data. If your data has the right context and uses a formal knowledge representation language, you can build very powerful applications on top of it, which enable you to go right to the question and visualization of interest without having to engage in data wrangling.
Q8. What specific data analytics techniques are most effective in life science R&D?
That’s a really broad question… but if you are asking about AI, we are already seeing that the advances in computer vision can have a real impact, for example for automated phenotype assessment of plants, or in digital pathology to support decision making in the hospital. In clinical drug development, you also see a trend where RCTs (randomized clinical trials) are increasingly complemented by large-scale analysis of so called ‘real world’ observational data from healthcare databases (see for example the OHDSI community). However, the biggest hurdle by far is the lack of interoperable data at scale. When we looked at possible applications for AI in drug discovery and medicine in the Pistoia Alliance, the lack of well-curated, high quality data was by far the biggest hurdle we consistently identified to advance this. In a podcast (OutsideVoices with Mark Bidwell) aired end of last year, Vas Narasimhan, the CEO of Novartis, commented that people underestimate how little clean data there really is to enable analytics and AI.
Q9. How do you mitigate the risk that people and/or processes create unwanted bias or incorrect results?
This again depends in the end on the intended use. In order to get value out of data integration using finite resources, you often have to take shortcuts and use a form of semantic integration which maps existing code systems to shared concepts. The FAIR principles establish the importance of always tracking the context provenance of your data: you should know what you are looking at and take that into account when you do your analysis. Doing the right thing vs doing it right applies here too: you should think about the question you are asking, as well as choose the datasets and algorithms you use to answer it carefully.
(*) Source: Kate Crawford, Keynote “The Trouble with Bias“Neural Information Processing System Conference.
————————-
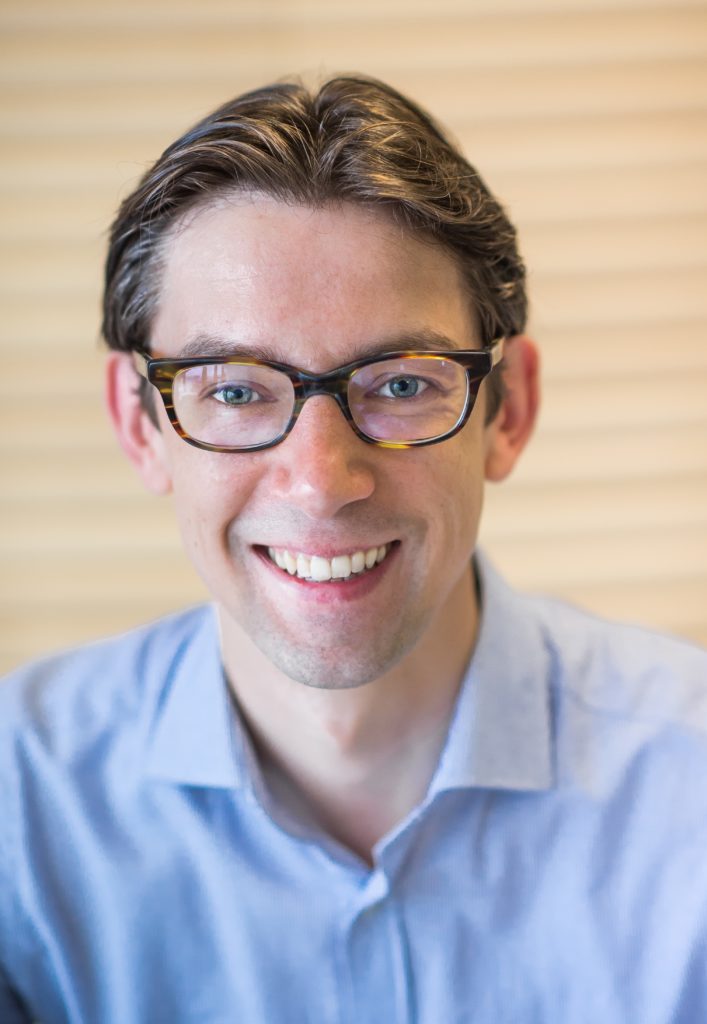
Kees studied Computer Science and Bioinformatics, for which he did his research project on lipoprotein metabolism at TNO Quality of Life in The Netherlands and the Jean Mayer USDA Human Nutrition Research Center on Aging at Tufts University, Boston. Through his many years of experience in open source software and standards development in bioinformatics, Kees has gained a deep understanding of all aspects of collaborative open source development. He founded The Hyve to facilitate open source software development in biomedical research.