On Generative AI. Interview with Maharaj Mukherjee
“Managing changes is one of the dimensions that an organization may adapt to for reaping the complete benefits out of Generative AI. It need to manage the change, adaptation and redeployment easy on its workforce so that people do not feel threatened by this new technology.”
Q1. Generative AI applications like ChatGPT, DALL-E, Stable Diffusion and others are said to rapidly democratizing the technology in business and society. Is this really happening ?
Mukherjee: Democratizing in the AI/ML area has been happening for some time. It is a slow evolutionary process. It started with the advent of auto ML tools whereby the model building moved very quickly from the expertise of data scientists to the purview of any one with a touch of a button. But except for some areas such as face recognition, etc., it has been out of reach for most people. Now with the coming of generative AI with large foundational models and large language models the doors have been opened to the public to experiment with AI in all different ways. But I do not think the ball will stop here and we are yet to see all the many ways people can make use of these new sets of tools that have suddenly become available to them. These use cases will now drive the development of newer types of research and innovation in the AI field.
Q2. What industries do you believe will be most impacted by LLMs and Generative AI? Why?
Mukherjee: In my humble opinion, the arts and entertainment industry as well as the advertisement industry would be the first adaptors of this technology and that is already happening. It will be happening slowly in the area that require more specialized knowledge such as in the Science, Technology, and Engineering and often more regulated such as healthcare and pharmaceutical industries.
Q3. What kind infrastructure will be essential for deploying generative AI?
Mukherjee: The current barrier to the early adaptation for any small industry are two folds. One is the availability of specialized hardware such as GPUs and the next is availability of quality data scientists and programmers who can make use of the best-known algorithms and best available hardware to make it work. These two limitations are keeping these technologies out of reach for most companies except for a few very large organizations. But I would think that it is a matter of time and with improvements of scales things will be within the reach of almost every organization.
Q4. How will companies prevent the breach of third-party copyright in using pre-trained foundation models?
Mukherjee: That is a main concern for existing LLM and Gen-AI models. However, many organizations are already in the process of building models based on Retrieval Augmented Generation (RAG) which can take care of copyright violation and other ethical and legal issues. Another way to handle such violations is tagging the generation and retrieval to the original source by keeping a record of all intermediate steps using methodologies such as block chain.
Q5. What about trust in generative AI? How can you ensure the accuracy of generative AI outputs and maintain user confidence?
Mukherjee: A model is always as good as the results it generates. The problem of errors is not new to the area of AI and ML and just calling it using some anthropomorphic terms such as “Hallucinations” does not in any case make it different. Often errors are introduced as a safety measure as a bias in the model. As more and more people get used to these fundamental limitations of AI, people will adjust their expectations and find how the best way to use these models.
Q6. LLMs may generate algorithmic bias due to imperfect training data or decisions implicitly or explicitly made by the engineers developing the models. What is your take on this?
Mukherjee: Quality of data has always been an issue with any AI/ML models, and it is not very different in the age of generative AI and LLM. It is always the case of “Garbage In – Garbage Out”. In traditional AI/ML model developments engineers have been culling and engineering data to suit their goal and need. But often the engineering bias sips in how the data is selected and consequently some human biases are introduced in the model. In the realm of Generative AI the philosophy is slightly different from traditional AI. Since it is built based on any and all kinds of data – the initial data bias may not be an issue here. However, we still need to make sure that the output follows our societal norms and principles and also not harmful in general.
Q7. Will change management be critical to implementing generative AI?
Mukherjee: Managing changes is one of the dimensions that an organization may adapt to for reaping the complete benefits out of Generative AI. It need to manage the change, adaptation and redeployment easy on its workforce so that people do not feel threatened by this new technology.
Q8. How is AI regulation going to have an impact when it comes to harnessing the opportunities of generative AI?
Mukherjee: As with any human technology Generative AI needs to conform to our accepted societal principles, norms, and moralities. If the technologist and the industry cannot regulate themselves, there is a risk that the regulations may be imposed upon them by outsiders who may not have as much knowledge and understanding of the technology. It is better, therefore that developers of Gen AI step back and spend some time figuring out how to do that and impose certain standard checks and balances upon themselves.
Q10. If it were up to you would you use generative AIi in mission-critical applications?
Mukherjee: I would repeat my thoughts as before that Gen AI is not fundamentally different from traditional AI. Any area where people have used any traditional AI in the past may consider adapting to Generative AI or at least explore the options as a possibility.
……………………………………….
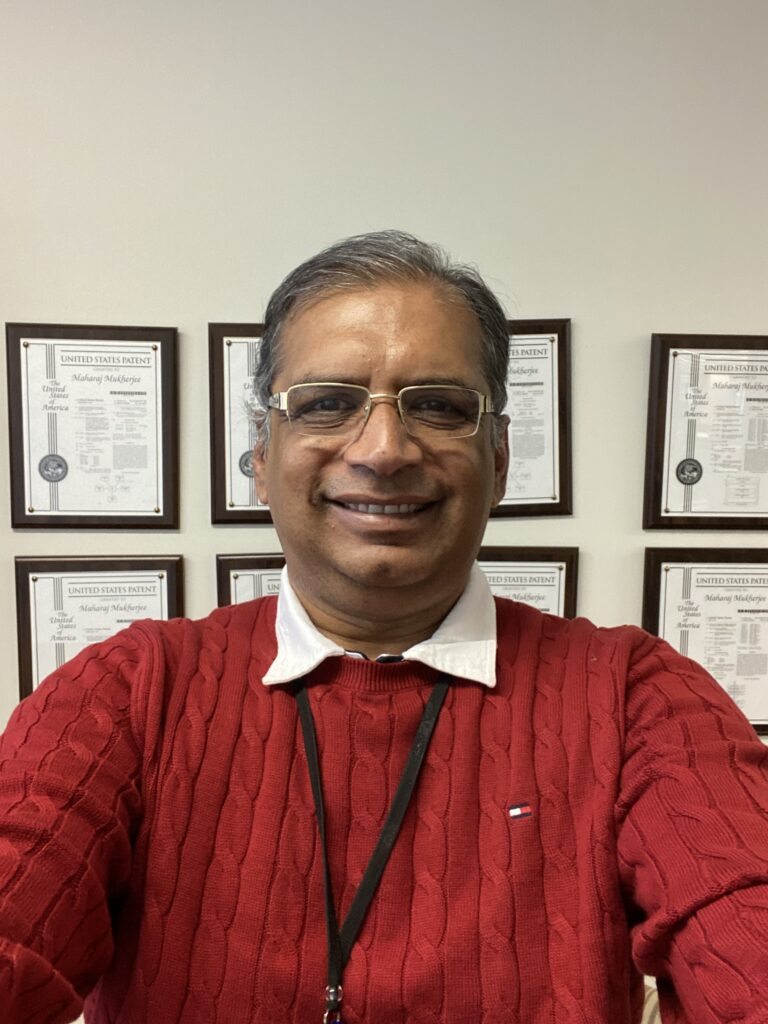
Maharaj Mukherjee, Senior Vice President and Senior Architect Lead, Bank of America
Well recognized expert in cutting edge technologies including Edge and Massively Distributed Computing, Artificial Intelligence and Machine Learning, Cognitive Deep Learning, Blockchain, and Internet-of-Things. Currently working in the Bank of America as Senior Vice President and Senior Architect Lead in the Technology Infrastructure Organization. Previously worked as Senior AI/ML Architect and SVP at the Employee Experience Technology within Bank of America. Before Bank of America Maharaj Mukherjee worked for twenty years in the IBM Research in various leading-edge technologies including Shape Processing Engine, Computational Lithography, Design for Manufacturing, Deep and Cognitive Machine Learning, and Watson Internet of Things. Maharaj Mukherjee is an IBM Master Inventor Emeritus and holds 162 US patents and 160 International Patents to his credit. He is also recognized as a top inventor in the Bank of America in 2020 and 2021. He received the Platinum Award from the Bank of America for being one of the top three inventors in 2021. He was also recognized by IBM for the “Twenty Patents from the Past Twenty years” in 2015. He was inducted in IBM’s Inventor Wall of Fame in 2011.
He holds a PhD from Rensselaer Polytechnic Institute, an MS from SUNY Stony Brook, and B-Tech (Hons.) from Indian Institute of Technology, Kharagpur.
He currently serves as a member of the Institute of Electrical and Electronics Engineers (IEEE) USA Awards Committee as well as a member of the IEEE Region 1 Awards Committee. He is also the current chair of Central Area of IEEE USA.
Related Posts
On Generative AI and Databases. Interview with Adam Prout, ODBMS Industry Watch, October 9, 2023
On Generative AI. Interview with Philippe Kahn, ODBMS Industry Watch, June 19, 2023
Follow us on X: @odbmsorg