On AI Factory and Generative AI. Interview with Ashok Reddy.
“ The AI Factory is a revolutionary concept aimed at streamlining the creation and deployment of AI models within organizations. It serves as a centralized hub where data scientists, engineers, and domain experts collaborate to develop, train, and deploy AI solutions at scale.“
Q1. You joined KX as Chief Executive Officer in August 2022 (*). How has the database and the AI market developed since then?
Ashok Reddy: Since taking on the role of Chief Executive Officer at KX in August 2022, I have observed significant transformations within the database and AI markets, underscored by burgeoning investments and innovations across several key segments:
Non-relational Databases (NRDBMSs): These databases have experienced notable growth, driven by the demand for flexible, scalable data management systems that accommodate the complex needs of modern, data-intensive applications.
Analytics and Business Intelligence Platforms: This segment has continued to expand rapidly, fueled by the increasing need for sophisticated analytical tools that can provide deeper insights into vast datasets, enabling more informed decision-making processes.
Data Science and AI Platforms: The emergence and integration of advanced data science and Generative AI (GenAI) technologies have propelled this sector forward, with organizations seeking powerful platforms that can drive AI-driven innovation and operational efficiency.
These industry segments have showcased annual growth rates ranging from 20 to 25%, highlighting a substantial shift towards technologies that are not only agile and scalable but also capable of underpinning advanced analytics and AI-driven applications.
Q2. It seems that securing a tangible return on investment from artificial intelligence (AI) is still a challenge. Do you agree? How can you ensure your AI has an ROI?
Ashok Reddy: Yes, achieving a tangible return on investment from artificial intelligence (AI) poses a challenge, but it’s not unattainable. AI, fundamentally a prediction technology as highlighted in “Power and Prediction” by Ajay Agrawal, Avi Goldfarb, and Joshua Gans, and “Competing in the Age of AI” by Karim R. Lakhani and Marco Iansiti, offers organizations the ability to make informed predictions based on extensive datasets. This capability is key to gaining a competitive advantage.
To ensure AI initiatives yield a tangible ROI, a strategic focus on leveraging prediction technology is crucial. This involves minimizing prediction costs, which can be achieved by reducing the incidence and impact of prediction errors and continually refining AI models to enhance their accuracy. By strategically lowering these costs, companies can boost operational efficiency, foster innovation, and elevate revenues.
Moreover, viewing AI’s predictive prowess as a strategic asset allows for the alignment of AI endeavors with specific business goals. Whether the aim is to streamline operational processes, improve customer experiences, or explore new market opportunities, the predictive capacity of AI can be effectively utilized to achieve concrete business results.
Q3. What is the AI Factory concept, and what is its significance?
Ashok Reddy: The AI Factory is a revolutionary concept aimed at streamlining the creation and deployment of AI models within organizations. It serves as a centralized hub where data scientists, engineers, and domain experts collaborate to develop, train, and deploy AI solutions at scale. The AI Factory is essential for organizations looking to leverage AI effectively across various business functions, enabling them to drive innovation, enhance productivity, and gain a competitive edge.
Q4. How does an AI Factory facilitate the utilization of a single GenAI model across multiple functions and tasks?
Ashok Reddy: An AI Factory empowers organizations to leverage a single GenAI model for a multitude of functions and tasks through systematic processes and automation. By centralizing model development and deployment, the AI Factory ensures consistency, scalability, and reusability of AI solutions. This enables organizations to streamline workflows, optimize resource allocation, and extract maximum value from their AI investments.
Q5. What are your tips for scaling AI in practice?
Ashok Reddy: Adopting an AI Factory approach will enable businesses to scale AI. In practice, this means changing how we think about the typical tasks our workforce is asked to complete and how we apply [AI] technology to support not only the completion of that task but the entire job motion itself. For example, if we can support paralegals to do the document review and preparation that a lawyer typically handles, we’re enabling that lawyer to dedicate more of their time to complex cases thereby revolutionizing the job processes for both sides.
By rethinking how we scale AI within the human workforce, we can empower professionals to pursue roles of greater strategic value, reduce time to market for AI solutions, and ensure consistency and quality across AI initiatives.
Q6. Let’s now look at GenAI. Several specialized companies offer so called GenAI “foundation models”– deep learning algorithms pre-trained on massive amounts of public data. How can enterprises take advantage of such foundation models?
Ashok Reddy: Foundation models provide a massive head start for enterprises, but their value lies in adaptation and thoughtful integration. Some examples of adoption of these models for organizations to consider are:
- Reducing Development Time and Costs: Instead of building complex AI models from scratch, enterprises can fine-tune foundation models for specific tasks, saving significant resources.
- Unlocking New Applications: The flexibility of foundation models (for text, image generation, etc.) enables creative applications, potentially disrupting existing workflows.
- Democratizing AI Access: Smaller businesses and those without in-house AI expertise can leverage pre-trained models via APIs or user-friendly platforms.
- The RAG Connection for Leveraging Private Data: Foundation models are the perfect ‘retrieval’ component of the retrieval-augmented generation (RAG) pipeline. Accessing knowledge at scale becomes easier.
However, to effectively deploy these strategies, enterprise organizations should consider putting a few guardrails in place, such as:
- Domain-Specific Fine-Tuning and Data: Introduce your own high-quality, domain-specific datasets to combat potential irrelevance or bias in the pre-trained model.
- Constraints and Sanity Checks: Embed rules derived from industry knowledge and common sense into your fine-tuned model to avoid unrealistic or undesirable outputs.
- Continuous Evaluation: Don’t treat a foundation model as static. Test regularly against real-world scenarios and adjust as needed
- Human-AI Collaboration: Emphasize explainability, critical evaluation of outputs, and maintaining human oversight. This is vital for trust and responsible deployment.
Q7. Three issues are very sensitive when talking about GenAI: quality and relevance of public data, ethics, and accountability. What is your take on this?
Ashok Reddy: I think these issues are important to consider as we, as a collective industry, work to create expanded capabilities in the area of GenAI.
When it comes to the quality and relevance of public data, the two most important considerations are bias amplification and domain mismatch. First, public datasets often contain biases. Enterprises need rigorous pre-processing and bias mitigation techniques to avoid harmful outputs. This should include work to identify and mitigate biases within the models during development and testing to make sure we’re approaching GenAI creation with a sense of fairness and inclusivity.
With regard to domain mismatch, it’s important to know that data used to train the foundation model may not align with your specific enterprise needs. Fine-tuning and supplementing with your own data is essential.
On the topic of ethics, we’re seeing instances of misinformation and deepfakes in our everyday consumption of news and social media. Organizations should ensure foundation models are not misused for malicious purposes by implementing safeguards and internal policies to guide responsible use. As a society, we need to consume with a discerning eye and make sure we hold creators accountable.
I believe that in the area of accountability, transparency, governance, and continuous monitoring of models are all critical issues to be addressed.
Where possible, aim for some level of transparency in your foundation model’s decisions. This builds trust and helps debug potential issues. Establish clear ownership and protocols for using GenAI within your enterprise, defining acceptable use cases and limitations.
And, as foundation models evolve, so too must your risk assessment and ethical considerations. Therefore, establishing a method for continuous monitoring is key.
To help accomplish oversight in these areas, it’s important that we adopt grounding strategies.
The first way to do this is to use your knowledge base as an anchor, supplementing public data with your curated knowledge base of reliable sources, financial reports, or industry-specific data. This helps the model gain a better understanding of your domain’s facts and principles.
Secondly, you should use RAG for verification. If your foundation model generates text, use a RAG model to cross-check its outputs against trusted knowledge sources, reducing the chance of spreading misinformation.
And finally, make sure to include explainability as a requirement. Prioritize techniques that give insight into why the model generates certain outputs. This helps spot issues and maintain a strong link to reality.
Q8. What staffing approach is required for operating an AI Factory? Is it about hiring new specialists or upskilling existing business and tech roles, or both?
Ashok Reddy: Operating a GenAI Factory requires a strategic approach to staffing that combines both hiring new specialists and upskilling existing talent. While recruiting individuals with expertise in AI, machine learning, and data science is crucial for driving innovation, it’s equally important to invest in upskilling existing business and tech roles. By fostering a culture of continuous learning and development, organizations can ensure that their workforce remains adaptable and proficient in leveraging advanced AI technologies effectively.
Q9. Harvard Business Review (**) reported that “Gartner research has identified five forces that will keep the pressure on executives to keep learning, testing, and investing in the GenAI technology: 1) Board and CEO expectations; 2) Customer expectations; 3) Employee expectations; 4) Regulatory expectations; and 5) Investor expectations.” What does it mean in practice?
Ashok Reddy: Boards and CEOs are looking to implement solutions that have clear data pipelines, experimentation processes and a focus on translating AI insights into actionable decisions, all attributes of a well-structured AI Factory for continuous innovation.
Customers want AI-enhanced experiences. Organizations can work to deploy AI from the Factory for personalized recommendations, intelligent chatbots, and streamlined processes. Constant evaluation and refinement are the keys to success.
When it comes to employees, using AI as a Co-pilot or Assistant can help upskill employees to collaborate with the AI tools developed in their AI Factory. This reduces mundane tasks and fosters a sense of empowerment.
When it comes to regulatory expectations, you can look to explainability and bias mitigation and work to design an AI Factory with transparency in mind. Incorporate tools and processes to explain AI outputs and proactively address potential biases in datasets and models.
Investors are going to look for an AI Factory to produce ROI. For this, organizations can demonstrate a clear link between their AI Factory investments and tangible business outcomes (cost savings, revenue growth, risk reduction), while also working to underscore their commitment to ethical and responsible AI use.
An AI Factory approach isn’t a magic solution but a strategic framework. Executives who develop a structured plan for building their AI Factory, one that is responsive to these five forces, will gain trust and secure sustained investment for AI initiatives.
Q10. How has KX developed since August 2022?
Ashok Reddy: Since August 2022, KX has been at the forefront of driving accelerated computing for data and AI-driven analytics, catering specifically to AI-first enterprises. Our focus on strategic partnerships has allowed us to deliver cutting-edge solutions tailored to meet the evolving needs of our customers.
Furthermore, our commitment to innovation and customer-centricity has solidified our position as a trusted partner in the AI-driven analytics space. By aligning our efforts with market demands, we continue to lead the way in delivering transformative solutions that drive success for our customers.
……………………………………….
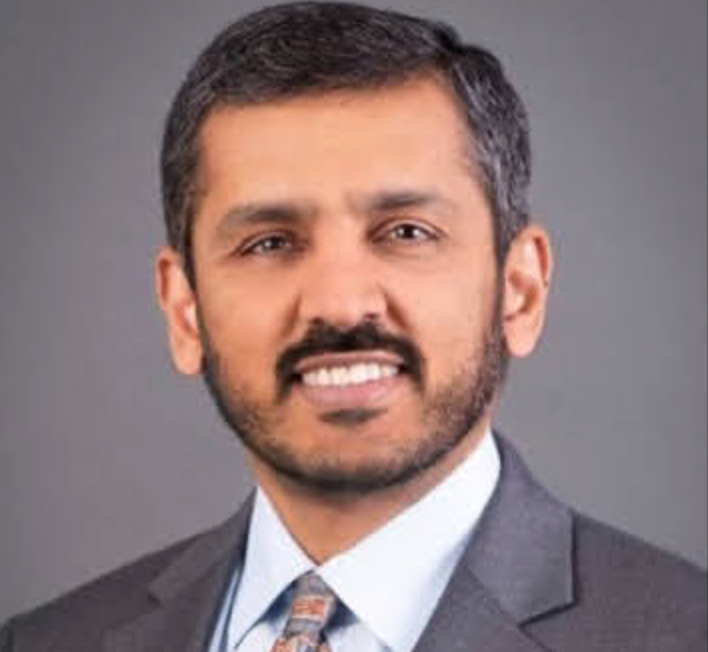
Ashok Reddy, CEO, KX
One of the leading voices in vector databases, search & temporal LLM’s, Ashok KX joined as Chief Executive Officer in August 2022. He has more than 20 years of experience leading teams and driving revenue for Fortune 500 and private equity-backed technology companies. He spent ten years at IBM as Group General Manager where he led the end-to-end delivery of enterprise products and platforms for a diverse portfolio of global customers. In addition, he has held leadership roles at CA Technologies and Broadcom, and worked as a special adviser to digital transformation company Digital.AI where he helped the senior leadership team devise the product and platform vision and strategy.
Resources:
(**) 5 Forces That Will Drive the Adoption of GenAI. Harvard Business Review, Dec 14, 2023.
Related Posts:
On Generative AI. Interview with Maharaj Mukherjee. ODBMS Industry Watch, December 10, 2023.
On Generative AI. Interview with Philippe Kahn. ODBMS Industry Watch, June 19, 2023.
…………………
Comments are closed.