“If a company starts as an on-premise business and decides to become relevant in the cloud space it requires dedicated energy devoted to changing the culture.
...If there was a lesson to share it would be to not under-invest or think it will be easy. You also need to hire people with cloud experience. There are a number of areas where you can hire smart people and they will pick up what they need, but if you’re trying to make a transition there is no substitute for actual hands-on experience with what happens when you try to scale.” — Jordan Tigani.
Q1. You are Chief Product Officer at SingleStore since June 30th, 2020. What are the main projects you have been working at SingleStore?
Jordan Tigani: The number one thing that I’ve been focused on is helping SingleStore transform into a cloud company. This means more than having a product that runs in the cloud; you need to reimagine how you build software, how you monitor it, how you support it, and what features you need. We’ve got a great team that has taken these ideas and ran with them, but to some extent, this is a cultural change, and that takes a lot of time and directed energy.
I’ve also been working on refining the mission and completing the technology so that it solves all use cases. For the last year, we’ve been focused on data-intensive applications, which are, broadly, applications that hit bottlenecks in data. This is a growing subset of the database market, as richer applications tend to want to do more interesting things with their data.
Q2. You co-created Google BigQuery as a founding engineer and went on to be the Director for Engineering Director and also Product Management. How much has your work at Google influenced your current work at SingleStore?
Jordan Tigani: My two biggest learnings from Google were how to build a cloud product that scales (when I left BigQuery it was using about 3 million CPU cores) and a deep customer empathy for the cloud analytics market.
I also saw a lot of things that customers wanted to do, but we had a hard time making the technology work to solve their problems. One of our tech leads had a great saying: “It’s just code.“ This meant that given enough time, you could make any feature work. However, if you didn’t have the right architecture you would hit limitations, and all the clever coding in the world would be able to help you.
Some of the things that BigQuery customers were pushing us to do—like being able to do rapid updates, or serve low latency queries—were things that were incredibly difficult to do with the architecture. Many of these same things were problems that SingleStore had already solved, and by virtue of their architecture, there was a technological moat that would be hard for competitors to cross.
Q3. The tag line of SingleStore is “The Single Database for All Data-Intensive Applications for operational analytics and cloud-native applications“. To demonstrate how fast SingleStore is on both transactional and analytical workloads you did comparative benchmarks against leading cloud vendors for both TPC-H (analytics) and TPC-C (transactions). What were the main results?
Jordan Tigani: The main takeaway was that SingleStore is as fast or faster on analytics benchmarks as cloud data warehouses, and is as fast or faster than cloud operational databases at transactional benchmarks. This means that in one database, with one storage type, you can get stellar performance on both transactions and analytics, which many people think is impossible. This brings us closer to having a “general purpose” database, where you don’t have to necessarily plan what you’re going to do with it before you start using it.
Q4. Why did you compare separately your performance at TPC-H with data warehouse vendors, and TPC-C with only one operational database vendor? What did you learn?
Jordan Tigani: On the analytics side, the main cloud data warehouse vendors have been engaging in public benchmark wars and focusing on performance. We didn’t want to escalate the amount of noise being thrown around, but we did want to call attention to the fact that we can put up some pretty stellar numbers ourselves.
We only measured one operational database vendor because TPC-C is harder to set up, and because of the way it is defined, it doesn’t provide rich information like TPC-H. We’re working with a third-party vendor to release a more detailed report, which will include additional operational database vendors.
Q5. How did your perform the test?
Jordan Tigani: We had ignored benchmarks for a long time since they often do not correlate with real-world performance. But in recent months, data warehouse vendors have been poking each other about TPC results. So, we put a couple of engineers on the problem and had them run some tests against our database and competitors.
When you run competitive benchmarks yourself you often get accused of selective reporting or cheating (Databricks and Snowflake had a recent public spat about this). We’ve hired a third-party vendor to reproduce the results and the report should be out in another month or so. When they do publish their report, they’ll also reveal the companies they are comparing us against.
Q6. You mentioned in the article that your benchmark runs used the schema, data and queries as defined by the TPC. However, they do not meet all the criteria for official TPC benchmark runs, and are not official TPC results. Isnt`t this a limitation to the acceptance of such bechnmarks?
Jordan Tigani: It is very expensive and difficult to do an official TPC submission, and at the end of the day, it doesn’t tell you much. For TPC-H, for example, we did a “power run,” which means running the queries sequentially. This shows off the ability to perform well in several different query shapes that are indicative of a data warehousing workload. It is a lot harder to run a full TPC-H benchmark as it involves multiple concurrent queries and changing data.
Q7. Not every workload needs transactions and analytics. What are the typical applications that need some flavor of both?
Jordan Tigani: There are two types of applications that need both analytics and transactions. The first is applications that are doing analytics. That is, they’re showing custom dashboards and slicing and dicing data. They tend to need up-to-the-moment data and low latency because they’re serving requests to end-users. They also need high concurrency because they are being used by analysts and are part of the end product being served. Data warehouses aren’t a great option in this use case, because they can’t scale to high concurrent user counts and are generally designed for throughput rather than low latency. SingleStore has a lot of customers in the financial services industry who back a lot of their portfolio analytics tools behind SingleStore databases.
The other type of application that needs analytics and transactions is one that wants to make use of data to enrich the experience. Maybe they want to do a product search and faceted drill-down. Maybe they want to show a leaderboard at a game. Traditional databases aren’t always great at these use cases once you get beyond a certain scale, and then performance can fall off a cliff. People end up stitching multiple databases together—maybe adding a cache on top of it because it is slow—and then have to deal with complexity to keep a consistent model and all the data in sync. Have you ever seen an application that showed a notification or unread message count, and then when you clicked on it there weren’t any notifications or unread messages? This is one of the ways this pattern shows up to the detriment of users; if they had used SingleStore they could keep those values in sync.
Q8. You are quoted saying that “Making the jump to being a cloud-native rather than just a company who runs their product on the cloud requires deep changes throughout the organization”. What are the key lessons learned you wish to share?
Jordan Tigani: If a company starts as an on-premise business and decides to become relevant in the cloud space it requires dedicated energy devoted to changing the culture. We drew up a 24-point score card last year and graded where SingleStore was on every axis of cloud readiness. The score card had everything from Elasticity to Auth to Scalability. We created a plan to get everything to “green” – it takes a long time and a lot of sustained energy, but it was worthwhile to do so.
It paid off considering we were one of the 20 databases recognized by Gartner in the 2021 Magic Quadrant for Cloud Database Management Systems. We believe that is something that could have not happened if we didn’t dedicate significant energy to making sure we were thoroughly cloud.
If there was a lesson to share it would be to not under-invest or think it will be easy. You also need to hire people with cloud experience. There are a number of areas where you can hire smart people and they will pick up what they need, but if you’re trying to make a transition there is no substitute for actual hands-on experience with what happens when you try to scale.
Q9. How is the pandemic changing the market for enterprise infrastructures?
Jordan Tigani: The pandemic is changing the market for enterprise infrastructure in two ways. First, it is accelerating the transition to the cloud. If you’ve got a physical server somewhere you have to have staff that physically maintains those machines, which goes in the opposite direction of a workforce that is becoming more distributed and remote in the pandemic.
Secondly, the pandemic is accelerating the need for fast, accurate data. If you’re in the office, you can often tell how things are going by the “buzz.” But if your only connection to your team and your customers is through zoom, there is a lot of key information that is missing. The only way to get some semblance of that information back is through data and being able to mine what customers are doing, how sales are going, and how much attrition you’re seeing in the workforce.
Big data analytics tools were, to some extent, developed to handle cases where you had so many customers that you couldn’t meet them all and could only get a pulse by looking at data. Google and Amazon are two companies that relied heavily on data because they had to. These techniques are being applied successfully when you may not have billions of customers, but have a difficult time reading their pulse.
Q10. Can you tell us a bit how did you help True Digital in Thailand to develop heat maps around geographies with large COVID-19 infection rates? What lessons did you learn?
Jordan Tigani: True Digital is a telecom provider in Thailand that was able to use cellular data to help track the spread of the pandemic. In the early days of Covid-19, there was a huge focus on getting answers quickly and they were able to build out and ship an application on top of SingleStore in a matter of weeks. One lesson we learned was that if you need to build something in a hurry that needs to scale quickly, making sure you have the right tools when you start is important. SingleStore was ideally suited for True Digital’s needs, and we helped them get something out faster than they would have otherwise. You can read more about our work with True Digital here.
Q11. You are quoted saying “I like the idea of using AI to augment and go beyond what you can do currently. There’s really intelligence, which is a step beyond analytics, which is driving real insight from the data and automatic insight from the data.” Can you please elaborate on this?
Jordan Tigani: There is a hierarchy of analytical needs, and at the base level is collecting data. If you don’t have the data, then you’re blind to what is happening in the data.
The next step is understanding the data sources, which requires a feedback loop with a human to understand what the data is telling you. Too often people try to skip this step and jump right making decisions based on the data, and they end up making the wrong decisions because the data isn’t actually telling them what they thought it was telling them. A great example I’ve seen of this is when people were looking at counts of customers, but every customer that wasn’t logged in got the same customer ID, so the averages got completely skewed.
Once you have data that is cleaned and reputable, you can start understanding what the data shows. This is where BI and dashboards come in. Insight tends to come from questions that someone asks, like “why were my sales down in the southern region?”
Where it starts to get interesting is when you take the next step; making data-driven decisions. You have data that you understand and rely on, and you have been able to drill down and ask questions. AI and machine learning can help you all along the way–from figuring out what data to capture, to the structure of your data, to answering questions. As the last step, you need absolute trust in the lower levels of the system, or else you risk making a lot of bad decisions that you can’t diagnose.
Q12. You are Board Member of Atlas Corps, whose mission is to address critical social issues.
Jordan Tigani: There are generally two types of organizations that address social issues: those that address the issues directly, and those that seek to address the roots of the problems. For example, an organization in the former category would help distribute food during a famine while the latter would help teach sustainable farming.
As an engineer and someone who appreciates the building of the right systems and architectures, organizations that help improve systems are most interesting to me. Atlas Corps generally goes one step further than just trying to address the root of problems; they seek to help train people who are themselves addressing the problem. Who are we to come in and tell people how to farm, for example? Why not help boost the people in those locations who already have the context, and help teach them how to build stronger and scalable organizations?
Q13. What are the current projects?
Jordan Tigani: The pandemic has been hard on Atlas Corps since their model involved bringing social sector leaders to the United States for training and service in social change organizations. If you can’t bring people into the country, or those organizations are working remotely, it’s difficult to make those programs work. Atlas Corps has been working on building out their model to handle remote work, at least partly, which has made it work and scale better during the pandemic. Their tagline is “talent is universal, opportunity is not,” which is a lesson I try to apply everywhere.
………………………………………………

Jordan Tigani, Chief Product Officer, SingleStore.
Jordan is the Chief Product Officer at SingleStore, where he oversees the engineering, product and design teams. He was one of the creators of Google BigQuery, wrote two books on the subject, and led first then engineering and then the product teams. He is the veteran of several star-crossed startups, and spent several years at Microsoft working on bit-twiddling.
Resources
TPC Benchmarking Results.Genevieve LaLonde, Jack Chen, Szu-Po Wang, SingleStore, 2022
Related Posts
Follow us on Twitter: @odbmsorg
“Time is a critical context for understanding how things function. It serves as the digital history for businesses. When you think about institutional knowledge, that’s not just bound up in people. Data is part of that knowledge base as well. So, when companies can capture, store and analyze that data in an effective way, it produces better results.” –Paul Dix.
Q1. InfluxData just announced accelerated IoT momentum with new customers and product features. Tell us what makes InfluxDB so well-suited to manage IoT data.
Paul Dix: We’re seeing time series data become vital for success in any industrial setting. The context of time is critical to understanding both historical and current performance. Being able to determine and anticipate trends over time helps companies drive improvements in mission-critical processes, making them more consistent, efficient and reliable. We built InfluxDB to facilitate every step of this process. We’ve been fortunate to work with several major players in the IIoT space already, so we’ve been able to really understand the workflows and processes that drive industrial operations and better develop solutions around them.
Q2. How do the new edge features for InfluxDB that you just announced help developers working with time series data for IoT and industrial settings?
Paul Dix: The new features give developers more flexibility and nimbleness in terms of architecture so that they can build more effective solutions on the edge that account for the resources they have available there. For example, we understand that some companies have very limited resources on the edge, so we’ve made it easier to intelligently deploy configurable packages there. By breaking down the stack into smaller components, developers can reduce the amount of software they need to install and run on the edge. At the same time, we want developers to have the option to do more at the edge if they can. That’s why we’ve made it easier to run analytics on persistent data at the edge and to replicate data from an edge instance of InfluxDB to a cloud instance.
We’re also working to make it easier for IoT/IIoT developers to manage the many devices that they need to deal with. One of our new updates allows developers to distribute processed data with custom payloads to thousands of devices all at once from a single script. On the other side of the equation, we have another new feature that helps contextualize IoT data generated from multiple sources, using Telegraf, our open source collection agent, and MQTT topic parsing.
Q3. What makes time series data so important for IoT and IIoT?
Paul Dix: Time is a critical context for understanding how things function. It serves as the digital history for businesses. When you think about institutional knowledge, that’s not just bound up in people. Data is part of that knowledge base as well. So, when companies can capture, store and analyze that data in an effective way, it produces better results. For example, manufacturers may want to know how long a valve has been in service, or how many parts their current configuration can produce per hour. Time is a constant measure that creates a baseline for comparative purposes, generates a current snapshot for systems and processes, and reveals a roadmap for identified patterns to persist and therefore become more predictable.
Time series data is well-suited to IoT and IIoT because it ties the readings from critical sensors and devices to the context of time. It’s also easy to use persistent time series data for multiple, different purposes. We can think about temperature in this case. In a consumer IoT context, such as a home thermostat, users primarily want to know what the current temperature is. In an IIoT context, manufacturers want to know the current temperature, but also what the temperature was in the last batch, or the batch from the previous week. Using InfluxDB to collect and manage time series data makes these kinds of tasks easy. At InfluxData, we’re fortunate that InfluxDB is one of a select group of successful projects and products where IoT, data, and analytics deliver significant value to organizations and the customers they serve.
Q4. Graphite Energy is featured in the announcement as a company that’s using InfluxDB to manage its time series data. Can you tell us more about the impact InfluxDB has had on its business?
Paul Dix: We’re really excited about our work with Graphite Energy – they’re an Australian company that makes thermal energy storage (TES) units. These devices get energy from renewable sources and store it until it’s required for industrial processes in the form of heat or steam. Its goal is to decarbonize industrial production.
All of Graphite Energy’s operations are grounded in data – they’re collecting time series data from their devices out in the field and use InfluxDB to store and analyze these millions of data points they’re collecting daily. Graphite Energy uses that data to optimize its products, to guide remote operation, engineering and reporting, and to inform product development and research vectors. InfluxDB has also been a key component in the development of their Digital Twin feature. For this, they use time series data to generate a real-time digital model of a TES unit, that is accurate to within five percent of actual device performance. This allows them to roll backward g and forward in time to track performance. The Digital Twin is a key component of the company’s predictive toolkit and ongoing product optimization efforts. The more efficient Graphite Energy’s TES units are, the better they’re able to facilitate decarbonization. That’s a win for everyone.
Q5. How are some of your other IoT customers using the InfluxDB platform?
Paul Dix: Our customers are doing great things in the IoT space. I’ll highlight just a few here quickly.
- Rolls-Royce Power Systems is using InfluxDB to improve operational efficiency at its industrial engine manufacturing facility. By collecting sensor data from the engines of ships, trains, planes, and other industrial equipment, Rolls-Royce is able to monitor performance in real time, identify trends, and predict when maintenance will be needed.
- Flexcity monitors and manages electrical devices for its customers. They also monitor supply-side energy output and use that information to dynamically shed or store excess electrical load in their monitored devices to help with grid balancing and demand response. They use InfluxDB as their managed time series platform. They use Flux to calculate complex, real-time metrics, and take advantage of tasks in InfluxDB for alerting and notifications.
- Loft Orbital: Using InfluxDB Cloud to collect and store IoT sensor data from its spacecrafts. The company flies and operates customer payloads with satellite buses, and uses InfluxDB to gain observability into its infrastructure and collect IoT sensor data, including millions of highly critical spacecraft metrics, with the business currently ingesting 10 million measurements every 10 minutes.
Q6. InfluxData has partnered with some of the leading manufacturing providers including PTC and Siemens. How have these partnerships benefitted shared customers?
Paul Dix: A lot goes into these partnerships on both ends, and we work really hard to make and keep them mutually beneficial. One thing that’s a real benefit to customers is when we’re able to integrate InfluxDB with our partner’s platform. Take PTC, for example. InfluxDB is the preferred time series platform for ThingWorx and there is a native integration within the PTC platform itself. That makes it a lot easier for customers to get up and running with InfluxDB, and because it’s already integrated with PTC, they know the two systems are going to play together nicely. Having a solution like that reduces a lot of time and stress that typically occurs in the development process, especially when building out new solutions or retrofitting old ones.
Beyond PTC, additional industry-leading IIoT platforms including Bosch ctrlX, Siemens WinCC OA, Akenza IoT and Cogent DataHub have also partnered with InfluxData to use InfluxDB as a supported persistence provider and data historian.
Q7. What’s on the horizon for InfluxData and InfluxDB this year? How do you plan to build on this momentum in IoT?
Paul Dix: IoT will continue to be a priority for our team this year. We’re also looking forward to bringing the benefits of InfluxDB IOx to InfluxDB users. InfluxDB IOx is a new time series storage engine that combines several cutting-edge open source technologies from the Apache Foundation. Written in Rust, IOx uses Parquet for on-disk storage, Arrow for in-memory storage and communication, and Data Fusion for querying. IOx focuses on boundless cardinality and high performance querying.
IoT and IIoT users will benefit from IOx since they will have the ability to use InfluxDB and its related suite of developer tooling for emerging operational use cases that rely on events, tracing, and other high cardinality data, along with metrics. We’re eager to integrate this project into our existing platform so our IoT users can monitor any number of assets without worrying about the volume or variety of their data.
The arrival of IOx to our cloud platform will enable IoT and IIoT users to store, query, and analyze higher precision data and raw events in addition to more traditional metric summaries. In addition to the real-time replication currently enabled from the edge with Telegraf and InfluxDB 2.0, IOx will enable bulk replication of Parquet files for settings where the edge may not have real-time connectivity. Users working with machine learning libraries in Python will find it easier to connect to and retrieve data at scale for training and predictions because of IOx’s support for Apache Arrow Flight.
Qx. Anything else you wish to add?
Paul Dix: The big takeaway is we’re really excited about the many applications for time series in IoT. Regardless of industry, time series is transforming our ability to understand the activities and output of people, processes and technologies impacting businesses. Nowhere is this more apparent than in IoT or industrial settings.
…………………………………………………….

Paul Dix is the creator of InfluxDB. He has helped build software for startups, large companies and organizations like Microsoft, Google, McAfee, Thomson Reuters, and Air Force Space Command. He is the series editor for Addison Wesley’s Data & Analytics book and video series. In 2010 Paul wrote the book Service-Oriented Design with Ruby and Rails for Addison Wesley’s. In 2009 he started the NYC Machine Learning Meetup, which now has over 7,000 members. Paul holds a degree in computer science from Columbia University.
Resources
Related Posts
On IoT and Time Series Databases. Q&A with Brian Gilmore. ODBMS.org, October 18, 2021.
Follow us on Twitter: @odbmsorg
##
“Today, AI can be a cluster bomb. Rich people reap the benefits while poor people suffer the result. Therefore, we should not wait for trouble to address the ethical issues of our systems. We should alleviate and account for these issues at the start.” — Ricardo Baeza-Yates.
Q1. What are your current projects as Director of Research at the Institute for Experiential AI of Northeastern University?
Ricardo Baeza-Yates: I am currently involved in several applied research projects in different stages at various companies. I cannot discuss specific details for confidentiality reasons, but the projects relate predominantly to aspects of responsible AI such as accountability, fairness, bias, diversity, inclusion, transparency, explainability, and privacy. At EAI, we developed a suite of responsible AI services based on the PIE model that covers AI ethics strategy, risk analysis, and training. We complement this model with an on-demand AI ethics board, algorithmic audits, and an AI systems registry.
Q2. What is responsible AI for you?
Ricardo Baeza-Yates: Responsible AI aims to create systems that benefit individuals, societies, and the environment. It encompasses all the ethical, legal, and technical aspects of developing and deploying beneficial AI technologies. It includes making sure your AI system does not interfere with a human agency, cause harm, discriminate, or waste resources. We build Responsible AI solutions to be technologically and ethically robust, encompassing everything from data to algorithms, design, and user interface. We also identify the humans with real executive power that are accountable when a system goes wrong.
Q3. Is it the use of AI that should be responsible and/or the design/implementation that should be responsible?
Ricardo Baeza-Yates: Design and implementation are both significant elements of responsible AI. Even a well-designed system could be a tool for illegal or unethical practices, with or without ill intention. We must educate those who develop the algorithms, train the models, and supply/analyze the data to recognize and remedy problems within their systems.
Q4. How is responsible AI different/similar to the definition of Trustworthy AI – for example from the EU High Level Experts group?
Ricardo Baeza-Yates: Responsible AI focuses on responsibility and accountability, while trustworthy AI focuses on trust. However, if the output of a system is not correct 100% of the time, we cannot trust it. So, we should shift the focus from the percentage of time the system works (accuracy) to the portion of time it does not (false positives and negatives). When that happens and people are harmed, we have ethical and legal issues. Part of the problem is that ethics and trust are human traits that we should not transfer to machines.
Q5. How do you know when an application may harm people?
Ricardo Baeza-Yates: This is a very good question as in many cases harm occurs in unexpected ways. However, we can mitigate a good percentage of it buy thinking in the possible problems before they happen. How exactly to do it is an area of current research, but already we can do many things:
- Work with the stakeholders of your system from the design to the deployment. That implies your power users, your non digital users, regulators, civil society, etc. They should be able to check your hypotheses, your functional requirements, your fairness measures, your validation procedures, etc. They should be able to contest you.
- Analyze and mitigate bias in the data (e.g., gender and ethnic bias), in the results of the optimization function (e.g., data bias is amplified or an unexpected group of users is discriminated) and/or in the feedback loop between the system and its users (e.g., exposure and popularity bias).
- Do an ethical risk assessment and/or a full algorithmic audit, that includes not only the technical part but also the impact of your system on your users.
Q6. What is your take on the EU proposed AI law?
Ricardo Baeza-Yates: Among the many details of the law, I think AI regulation poses two significant flaws: First, we should not regulate the use of technology but focus instead on the problems and sectors in a way that is independent of the technology. Rather than restrict technology that may harm people, we can approach it the same as food or health regulations that work for all possible technologies. Otherwise, we will need to regulate distributed ledgers or quantum computing in the near future.
The second flaw is that risk is a continuous variable. Dividing AI applications into four risk categories (one is implicit, the no risk category) is a problem because those categories do not really exist (see The Dangers of Categorical Thinking.) Plus, when companies self-evaluate, it presents a conflict of interest and a bias to choose the lowest risk level possible.
Q7. You mentioned that “we should not regulate the use of technology, but focus instead on the problems and sectors in a way that is independent of the technology”. AI seems to introduce an extra complexity, that is, the difficulty in many cases to explain the output of an AI system. If you are making a critical decision that can affect people based on an AI algorithm for which you do not know why it produced an output, it would be in your analogy equivalent to allow a particular medicine to be sold that is producing lethal side effects. Do we want this?
Ricardo Baeza-Yates: No, of course not. However, I do not think it is the best analogy, as the studies needed for a new medicine must find why the side effects occur and after that you do an ethical risk assessment to approve it (i.e., the benefits of the medicine justify the lethal side effects). But the analogy is better for the solution. We may need something similar to the FDA in the U.S.A. that approves each medicine or device via a 3-phase study with real people. Of course, this is needed only for systems that may harm people.
Today, AI can be a cluster bomb. Rich people reap the benefits while poor people suffer the result. Therefore, we should not wait for trouble to address the ethical issues of our systems. We should alleviate and account for these issues at the start. To help companies confront these problems, I compiled 10 key questions that a company should ask before using AI. They address competence, technical quality, and social impact.
Q8. Ethics principles have been established long ago, well before AI and new technology were invented. Laws are often running behind technology, and that is why we need ethics. Do you agree?
Ricardo Baeza-Yates: Ethics always runs behind technology too. It happened with chemical weapons in World War I and nuclear bombs in World War II, to mention just two examples. And I disagree because ethics is not something that we need, ethics is part of being human. It is associated with feeling disgust, when you know that something is wrong. So, ethics in practice existed before the first laws. Is the other way around, laws exist because there are things so disgusting (or unethical) that we do not want people doing them. However, in the Christian world, Bentham and Austin proposed the separation of law and morals in the 19th century, which in a way implies that ethics applies only to issues not regulated by law (and then the separation boundary is different in every country!). Although this view started to change in the middle of the 20th century, the separation still exists, which for me does not make much sense. I prefer the Muslim view where ethics applies to everything and law is a subset of it.
Q9. A recent article you co-authored “is meant to provide a reference point at the beginning of this decade regarding matters of consensus and disagreement on how to enact AI Ethics for the good of our institutions, society, and individuals.” Can you please elaborate a bit on this? What are the key messages you want to convey?
Ricardo Baeza-Yates: The main message of the open article that you refer to is freedom for research in AI ethics, even in industry. This was motivated by what happened with the Google AI Ethics team more than a year ago. In the article we first give a short history of AI ethics and the key problems that we have today. Then we point to the dangers: losing research independence, dividing the AI ethics research community in two (academia vs. industry), and the lack of diversity and representation. Then we propose 11 actions to change the current course, hoping that at least some of them will be adopted.
……………………………………………………………

Ricardo Baeza-Yates is Director of Research at the Institute for Experiential AI of Northeastern University. He is also a part-time Professor at Universitat Pompeu Fabra in Barcelona and Universidad de Chile in Santiago. Before he was the CTO of NTENT, a semantic search technology company based in California and prior to these roles, he was VP of Research at Yahoo Labs, based in Barcelona, Spain, and later in Sunnyvale, California, from 2006 to 2016. He is co-author of the best-seller Modern Information Retrieval textbook published by Addison-Wesley in 1999 and 2011 (2nd ed), that won the ASIST 2012 Book of the Year award. From 2002 to 2004 he was elected to the Board of Governors of the IEEE Computer Society and between 2012 and 2016 was elected to the ACM Council. Since 2010 is a founding member of the Chilean Academy of Engineering. In 2009 he was named ACM Fellow and in 2011 IEEE Fellow, among other awards and distinctions. He obtained a Ph.D. in CS from the University of Waterloo, Canada, in 1989, and his areas of expertise are web search and data mining, information retrieval, bias and ethics on AI, data science and algorithms in general.
Regarding the topic of this interview, he is actively involved as expert in many initiatives, committees or advisory boards related to Responsible AI all around the world: Global AI Ethics Consortium, Global Partnership on AI, IADB’s fAIr LAC Initiative (Latin America and the Caribbean), Council of AI (Spain) and ACM’s Technology Policy Subcommittee on AI and Algorithms (USA). He is also a co-founder of OptIA in Chile, a NGO devoted to algorithmic transparency and inclusion, and member of the editorial committee of the new AI and Ethics journal where he co-authored an article highlighting the importance of research freedom on ethical AI.
…………………………………………………………………………
Resources
–AI and Ethics: Reports/Papers classified by topics
-– Ethics Guidelines for Trustworthy AI. Independent High-Level Expert Group on Artificial Intelligence. European commission, 8 April, 2019. Link to .PDF
– WHITE PAPER. On Artificial Intelligence – A European approach to excellence and trust. European Commission, Brussels, 19.2.2020 COM(2020) 65 final. Link to .PDF
– Proposal for a REGULATION OF THE EUROPEAN PARLIAMENT AND OF THE COUNCIL LAYING DOWN HARMONISED RULES ON ARTIFICIAL INTELLIGENCE (ARTIFICIAL INTELLIGENCE ACT) AND AMENDING CERTAIN UNION LEGISLATIVE ACTS. LINK
– Recommendation on the ethics of artificial intelligence. UNESCO, November 2021. LINK
– Recommendation of the Council on Artificial Intelligence. OECD,22/05/2019 LINK
–– How to Assess Trustworthy AI in practice, Roberto V. Zicari, Innovation, Governance and AI4Good, The Responsible AI Forum Munich, December 6, 2021. DOWNLOAD .PDF: Zicari.Munich.December6,2021
Related Posts
– On Responsible AI. Interview with Kay Firth-Butterfield, World Economic Forum. ODBMS Industry Watch. September 20, 2021
Follow us on Twitter: @odbmsorg
“People are our biggest asset, and we have been continually investing in and advancing our People digital and data science capabilities.” –Sastry Durvasula.
I sat down with Sastry Durvasula, Global Chief Technology & Digital Officer, and John Almasan, Distinguished Engineer, Technology & Digital Leader, at McKinsey to learn how the firm is leveraging AI, cloud, and data & analytics to power digital colleague experiences and client service capabilities in the new normal of hybrid work.
RVZ
Q1: Can you explain the role of technology and digital capabilities at McKinsey? What is your strategy for advancing the firm in the new normal?
SD: The firm has experienced significant growth over the last few years, with nearly 40K colleagues serving clients across 150 global locations. Our technology and digital strategy is focused on powering the future of the firm with a range of innovative capabilities, platforms, and experiences. Our strategic shifts include doubling our innovation in digital client service, firm-wide cloud transformations of all our platforms and applications, next-gen capabilities for AI and knowledge management, and leading-edge colleague-facing technology and hybrid experiences.
As per our recent study, Cloud is a trillion dollar opportunity for businesses, and we are very actively working with our clients to advance their cloud journey. Earlier this year, we acquired cloud consultancy Candid and their accomplished team of 100+ technical experts, helping us accelerate our clients’ end-to-end cloud transformations.
5K+ technologists at the firm are organized across our global guilds, which include Design, Product Management, Engineering & Architecture, Data Science, Cyber, etc., and they provide digital transformation solutions to our clients and development of assets and internal capabilities. Our agile Ways of Working (WoW) and build-buy-partner models are central to our product development, empowering teams to innovate at speed and scale, with psychological safety to experiment and learn.
Q2: What roles do cloud, data science, and AI play in your strategy? Can you provide some examples?
SD: AI and data science are central to this strategy in both serving our clients and transforming our internal capabilities. Thanks to the significant technological advancements in AI/ML powering our data science capabilities, we are unlocking innovative client-service and colleague digital experiences. We are building and advancing a hybrid and multi-cloud ecosystem to power distinctive solutions and assets for our clients, which includes strategic partnerships and integrations with leading industry hyperscalers and software products.
As an example, on the client-service side, we are completely transforming our core knowledge and expertise platforms leveraging cloud-native technologies and AI/ML. Similarly, McKinsey.com and the McKinsey Insights mobile app serve up strategic insights, analytics, studies, and content to a broad range of users across the globe — including the C-suite and aspiring students alike. Our cloud transformation of these iconic platforms enables innovation, scale, and speed in publishing, smart search, audience engagement, subscriber experience, and reach & relevance efforts.
On the colleague experience side, AI and AR/VR powered digital workplace capabilities, colleague-facing chatbots, and hybrid-in-a-box tools are a huge focus, as well as predictive and proactive services to detect and service technology issues for our global workforce. People analytics, recruiting, and onboarding journeys are also key areas where we are leading with distinctive capabilities and tools supported by data and AI-driven HR, allowing us to achieve a substantial step up from HR 2.0 to HR 3.0.
Q3: Can you elaborate on knowledge and expertise management, and the role AI plays in shaping this space at the firm?
SD: We have a unique and proprietary knowledge management platform that codifies decades of wisdom and integrates the firm’s extensive insights, studies, industry domain content, knowledge, structured and unstructured data, and analytics with a wide range of artifacts using secure and role-based access. This platform is widely used by our colleagues across the globe, creating profound impact to our clients as well as our firm’s business functions. We have been advancing this platform and the surrounding ecosystem by leveraging AI and cloud technologies for semantic searches, auto-curated and personalized results. Important to mention are our AI-powered chatbots with NLP, which provide valuable intelligence for our colleagues in various industry practices. Using graph database technologies and data science modeling for contextual understanding significantly enhances our knowledge search capabilities, including video scanning, speech to text, summarization, and the ability to index topics of interest.
For finding expertise, we are also making use of ML ontologies to uncover behaviors and relationships between various types of “skills” and Subject Matter Experts (SMEs) to manage, govern, and dynamically connect colleagues with the best domain experts based on desired skills and/or knowledge needs. Our colleague-facing “Know” mobile app provides on-the-go access to our curated knowledge databases and domain experts, integrating with all our internal communication channels and collaboration tools, and AI-driven recommendations.
Q4: Can you expand a little bit more on how AI and data science are powering the HR 3.0 agenda?
SD: People are our biggest asset, and we have been continually investing in and advancing our People digital and data science capabilities. For example:
People analytics play a vital role, and we consider them a stairway to impact with growing maturity in data, engineering, and data science capabilities. Our transformation to HR 3.0 relies on globally rich datasets, cloud capabilities, advanced analytics, and first-class data science and engineering teams, along with integrated operational processes. By making use of hybrid cloud-based graphs databases, R, Python, Julia, etc. to join disparate sources of data, our data engineering teams assemble not only one of the highest data quality ecosystems in the firm, but also a very resilient one. Being aware of the fact that, in general, 80% of data science effort is with data cleaning, our strategy removes such roadblocks and ensures an analytically ready, understandable data solution, so our data scientists can be effective in delivering people analytics rather than data curation and sanitization.
On the recruiting and onboarding front, given our scale of hiring talent every year across the globe — both fresh talent from innovative academic institutions and experienced hires from various industries with a wide range of skills— we have significantly invested in AI-driven capabilities for identifying, recruiting, and hiring talented individuals. As an example, our intelligent NLP driven “Resume Processing Review,” built with the use of deep learning models, enables us to process over 750,000 resumes annually and to identify characteristics of successful applicants. By making use of intelligent guidance with dynamic customizable questions, activities like scoring, prioritizing, and sorting candidates are simplified while the overall process timeline is tremendously reduced. Ensuring that solutions avoid AI bias in recruiting is also a major focus. Additionally, these AI capabilities are beneficial for enabling a smooth and personalized onboarding experience for candidates.
Our recent report the workforce of the future highlights the emerging trends and insights, which include flexibility and continuous learning opportunities to foster and retain an engaged workforce. Our “Job-to-Job Matching” ML system accelerates the discovery and matching of jobs with those looking for another opportunity. AI-driven learning is another big priority, which enables highly personalized learning tracks for our colleagues based on their skills, engagements, and aspirations as part of our proprietary platforms.
Q5: Can you share some insights and details on your technology ecosystem and how it powers your internal and external platforms and products?
JA: To power our global product development solutions and innovations, we focused on transforming the firm’s core technology architecture with a more robust yet flexible 7-layer stack. This new framework is based on hybrid and multi-cloud platforms, secure-by-design engineering capabilities, and futuristic tools to propel delivery at scale and speed.
Developer experience is a core focus, providing premium software engineering tools, APIs, and services across hyperscalers. Our modularized platform as a service, consolidated into a service catalog, allows developers the flexibility and agility to customize complex computing and infrastructure designs to address any internal and external tech ecosystem. Our AI driven CI/CD pipeline enables interoperability across a wide range of technologies, and identifies in real-time SDLC vulnerabilities to reduce potential risk and improve the overall software quality. Data scientists play a vital role across a range of studies and client-service. The stack includes a specialized studio for data scientists with state-of-the-art MLOps and AIOps tools and libraries.
We have developed a cloud security framework, enabling our E2E solutions to be built with secure-by-design and “zero trust” principles in mind, meeting or exceeding the industry “security posture” standards and regulatory needs. Lastly, our global presence demands proactive planning and innovative technologies to ensure that our internal and external platforms and products exist in ecosystems that comply with various country and region-level regulations.
Q6: Tell me about how AI powered colleague experiences helped during the pandemic.
JA: Digital colleague experience has been more crucial than ever during the pandemic and in a hybrid world. We are employing AI to enable seamless capabilities, tools, and rapid response time to client-service requests and issue handling.
First, let me start with CASEE (Caring And Smart Engineered Entity), our colleague-facing chatbot, which provides intelligent technology support and services across the globe. CASEE leverages conversational NLP, leading open source frameworks, and off-the-shelf tool integrations with the ability to improvise from every interaction and support request. It has been a huge help during the pandemic, when our global workforce switched to remote with an unprecedented spike in demand while we were also dealing with the effects on our global servicing teams. As an example, CASEE was specifically trained in less than a week to respond and handle 90% of the questions regrading remote working and common device and network issues. It has also been integrated with our digital collaboration tools as well as incident response systems.
Another example is the intelligent automation of our Global Helpdesk capabilities, which we turbo-charged during the pandemic and are widely recognized in the industry and by our clients as a go and see reference. We’ve augmented our tools with AI driven services that can intelligently detect hardware and/or software deterioration on our users’ machines and can proactively fix or mitigate these problems. The system is capable of initiating a laptop replacement, perform driver updates, trigger software patching, or even remove or stop glitched software.
Q7: I heard about the firm’s open source efforts. Can you elaborate?
JA: We recognized the fact that McKinsey tech has a great opportunity to support and to give back to the Open Source Community. Kedro, for example, is a powerful ML framework for creating reproducible, maintainable, and modular data science code. It seamlessly blends software engineering concepts like modularity, separation of concerns, and versioning, and then applies them to ML code. Kedro proved to be one of our most valuable ML solutions, and it was successfully used across more than 50 projects to date, providing a set of best practices and a revolutionized workflow for complex analytics projects. We’ve open-sourced Kedro to support both our clients and non-clients alike, and to foster ML and software engineering innovation within the community of developers. Our approach starts with our global guilds first, and then contributing to open source. Stay tuned for more exciting developments in this space.
Q8: How are you attracting and developing talent in this highly competitive market?
SD: As you can see, we have some very exciting and interesting problems across a wide-range of technologies, industries, geographies, and next horizon initiatives. We are constantly focused on attracting inquisitive and continuous learners. We have also been fostering deep strategic relationships with universities and industry networks across the globe.
We have been expanding our global hubs, adding new locations and advancing our hybrid/remote workforce capabilities across the US, Europe, Asia, and Latin America with several hundred active open jobs as we speak. We are also opening a major new center in Atlanta, which will be home to more than 600 technologists and professionals, and with strong diversity, inclusivity, and sense of community. We are partnering with leading non-profits including Girls in Tech globally, Chzechitas in Prague, and Black Girls Code and Historically Black Colleges and Universities (HBCUs) in the US.
We launched personalized development programs for our colleagues, including certifications in cloud, cyber, and other emerging technologies. Over 60% of our developers are certified in one or more cloud ecosystems. We’re proud of being recognized by Business Insider as one of the 50 most attractive employers for engineering and technology students around the world. At #19, we are the highest-ranked professional services firm on the list.
……………………………………………
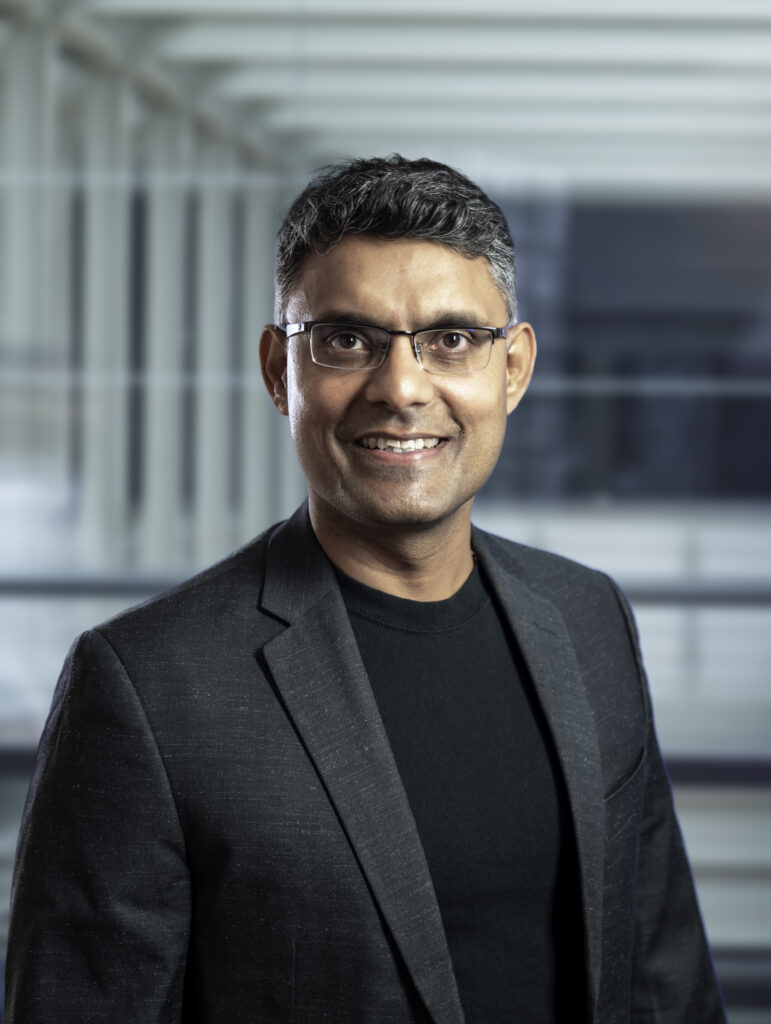
Sastry Durvasula is the Global Chief Technology and Digital Officer, and Partner at McKinsey. He leads the strategy and development of McKinsey’s differentiating digital products and capabilities, internal and client-facing technology, data & analytics, AI/ML and Knowledge platforms, hybrid-cloud ecosystem, and open-source efforts. He serves as a senior expert advisor on client engagements, co-chairs the Firm’s technology governance board, and leads strategic partnerships with tech and digital companies, academia, and research groups.
Previously, Sastry held Chief Digital Officer, Chief Data & Analytics, CIO, and global technology leadership roles at Marsh and American Express and worked as a consultant at Fortune Global 500 companies, with a breadth of experience in the technology, payments, financial services, and insurance domains.
Sastry is a strong advocate for diversity, chairs DE&I at McKinsey’s Tech & Digital, and is on the Board of Directors for Girls in Tech, the global non-profit dedicated to eliminating the gender gap. He championed industry-wide initiatives focused on women in tech, including #ReWRITE and Half the Board. He holds a Master’s degree in Engineering, is credited with 30+ patents, and has been the recipient of several honors and awards as an innovator and industry influencer.

John Almasan is a Distinguished Engineer, Technology & Digital Leader at McKinsey. He is a hands-on, accomplished technology executive with 20+ years of experience in leading global tech teams and building large-scale data, analytics, and cloud platforms. He has deep expertise in hybrid multi-cloud big data engineering, machine learning, and data science. John is currently focused on engineering solutions for the firm’s transformation and the build of the next gen data analytics platform.
Previously John held engineering leadership roles with Nationwide Insurance, American Express, and Bank of America focusing on cloud, data & analytics, AI and ML in financial services and insurance domains. He gives back through his pro bono consultancy work for the Arizona Counterterrorism Center, the Rocky Mountain Information Center, and as a member of the Arizona State University’s Board of Advisors.
John holds a Master’s degree in Engineering, a Master of Public Administration, and a Doctor of Business Administration. He is an AWS Educate Cloud Ambassador, Certified AWS Data Analytics & ML engineer, GCP ML Certified. John is credited with 10+ patents and has been the recipient of several awards.
Resources
The state of AI in 2021– December 8, 2021 | Survey. The results of our latest McKinsey Global Survey on AI indicate that AI adoption continues to grow and that the benefits remain significant— though in the COVID-19 pandemic’s first year, they were felt more strongly on the cost-savings front than the top line. As AI’s use in business becomes more common, the tools and best practices to make the most out of AI have also become more sophisticated
The search for purpose at work, June 3, 2021 | Podcast. By Naina Dhingra and Bill Schaninger. In this episode of The McKinsey Podcast, Naina Dhingra and Bill Schaninger talk about their surprising discoveries about the role of work in giving people a sense of purpose. An edited transcript of their conversation follows.
Related Posts
Follow us on Twitter: @odbmsorg
##
On Designing and Building Enterprise Knowledge Graphs. Interview with Ora Lassila and Juan Sequeda
“The limits of my language mean the limits of my world.” – Ludvig Wittgenstein
I have interviewed Ora Lassila, Principal Graph Technologist in the Amazon Neptune team at AWS and Juan Sequeda, Principal Scientist at data.world. We talked about knowledge graphs and their new book.
RVZ
Q1. You wrote a book titled “Designing and Building Enterprise Knowledge Graphs”. What was the main motivation for writing such a book?
Ora Lassila and Juan Sequeda: We wanted to tackle the topic of knowledge graphs more broadly than just from the technology standpoint. There is more than just technology (e.g., graph databases) when it comes to successfully building a knowledge graph.
Time and time again we see people thinking about knowledge graphs and jumping to the conclusion that they just need a graph database and start there. Not only is there more technology you need, but there are issues with people, processes, organizations, etc.
Q2. What are knowledge graphs and what are they useful for?
Ora Lassila and Juan Sequeda: We see knowledge graphs as a vehicle for data integration and to make data accessible within an organization. Note that when we say “accessible data”, we really mean this: accessible data = physical bits + semantics. The semantics part is really important, since no data is truly accessible unless you also understand what the data means and how to interpret it. We call this issue the “knowledge/data gap”; Chapter 1 of our book gets deep into this.
You could say that knowledge graphs are a way to “democratize” data: make data more accessible and understandable to people who are not technology experts.
Q3. Why connecting relational databases with knowledge graphs?
Ora Lassila and Juan Sequeda: Frankly, the majority of enterprise data is in relational databases, so this seemed like a very good way to scope the problem. At the beginning of our book we show examples of how data is connected today and frankly, it’s a pain. And it’s not just a technical pain, there are important social and organizational aspects to this.
Juan Sequeda: Understanding the relationship between relational databases and the semantic web/knowledge graphs has been my quest since my undergraduate years. The title of my PhD dissertation is “Integrating Relational Databases with the Semantic Web”. Therefore I can say that this is a passion of mine.
Q4. Does it make more sense to use a native graph database instead or a NoSQL database?
Ora Lassila and Juan Sequeda: There is always the question “why use X instead of Y?”… and the answer almost always is “it depends”. We even bring this up in the foreword: As computer scientists we understand that there are many technologies that can be used to solve any particular problem. Some are easier, more convenient, and others are not. Just because you can write software in assembly language does not mean you shouldn’t seek to use a high-level programming language. Same with databases: find one that suits your purpose best.
Q5. What are the typical roles within an organization responsible for the knowledge graph?
Ora Lassila and Juan Sequeda: Organizations really need to get into the mindset of treating data as a product. When you acknowledge this, you realize you need the roles for designing, implementing and managing products, in this case data products. We see upcoming roles such as data product managers and knowledge scientists (i.e. Knowledge Engineers 2.0). We get into this in Chapter 4 of our book.
Q6. Data and knowledge are often in silos. Sharing knowledge and data is sometimes hard in an enterprise. What are the technical and non technical reasons for that?
Ora Lassila and Juan Sequeda: Technical problems are solvable, and many solutions exist. That said, we think knowledge graphs are really addressing this issue nicely.
The non-technical issues are an interesting challenge, and in many ways more difficult: people and process, organizational structure, centralization vs decentralization, etc. One specific issue that shows up all the time is this: If you want to share knowledge within a broader organization, you have to cross organizational boundaries, and that lands you on someone else’s “turf”. There is a great deal of diplomacy that is needed to tackle these kinds of issues.
Q7. When is it more appropriate to use RDF graph technologies instead of native property graph technologies?
Ora Lassila and Juan Sequeda: First, we object to the notion of “native” when it comes to property graphs, they are no more native than RDF graphs.
These are two slightly different approaches to building graphs. Ultimately, the question is not all that interesting. A more interesting question is: When should you use a graph as opposed to something else? If you do decide to use a graph, there are a lot of considerations and modeling decisions before you even come to the question of RDF vs. property graphs.
Of course, RDF is better suited to some situations (e.g., when you use external data, or have to merge graphs from different sources). Try using property graphs there and you merely end up re-inventing mechanisms that are already part of RDF. On the other hand, property graphs often appeal more to software developers, thanks to available access mechanisms and programming language support (e.g., Gremlin).
Q8. How can enterprises successfully adopt knowledge graphs to integrate data and knowledge, without boiling the ocean?
Ora Lassila and Juan Sequeda: First of all, you can’t build enterprise knowledge graphs in a “boil the ocean” approach. No chance in hell. You first need to break the problem in smaller pieces, by business units and use cases. This ultimately is a people and process problem. The tech is already here.
That said, there is a certain “build it and they will come” aspect to knowledge graphs. You should think of them more as a platform rather than as an application. Start by knowing some use cases, and gradually generalize and widen your scope. But you need to be solving some pressing problems for the business. Spend time understanding the problems, the limitations of their current solutions (assuming they are somewhat viable) and finding a champion (i.e. “if you can solve this problem better/faster/etc, I’m all ears!”). Also try to avoid educating on the technology: Business units don’t care if their problem is solved with technology A, B or C… all they want is for their problem to be solved.
Q9. Knowledge graphs and AI. Is there any relationships between them?
Ora Lassila and Juan Sequeda: Yes. Knowledge Graphs are a modern solution to a long-time (and in some ways, “ultimate”) goal in computer science: to integrate data and knowledge at scale. For at least the past half century, we’ve seen independent and integrated contributions coming from the AI community (namely knowledge representation, a subfield of classical AI) and the data management community. See section 1.3 of the book.
Qx Anything else you wish to add?
Ora Lassila and Juan Sequeda: We see a lot of what Albert Einstein gave as the definition of insanity: Doing the same thing over and over, and expecting different results. We need to do something truly different. But this is challenging for many reasons, not least because of this:
“The limits of my language mean the limits of my world.” – Ludvig Wittgenstein
For example, if SQL is your language, it may be very hard for you to see that there are some completely different ways of solving problems (case in point: graphs and graph databases).
Another challenge is that there are hard people and process issues, but as technologists we are wired to focus on technology, and to seek how to scale and automate.
Finally, we think the “graph industry” needs to evolve past the RDF vs. property graphs issue. Most people do not care. We need graphs. Period.
………………………………………..

Dr. Ora Lassila, Principal Graph Technologist in the Amazon Neptune team at AWS, mostly focusing on knowledge graphs. Earlier, he was a Managing Director at State Street, heading their efforts to adopt ontologies and graph databases. Before that, he worked as a technology architect at Pegasystems, as an architect and technology strategist at Nokia Location & Commerce (aka HERE), and prior to that he was a Research Fellow at the Nokia Research Center Cambridge. He was an elected member of the Advisory Board of the World Wide Web Consortium (W3C) in 1998-2013, and represented Nokia in the W3C Advisory Committee in 1998-2002. In 1996-1997 he was a Visiting Scientist at MIT Laboratory for Computer Science, working with W3C and launching the Resource Description Framework (RDF) standard; he served as a co-editor of the RDF Model and Syntax specification.
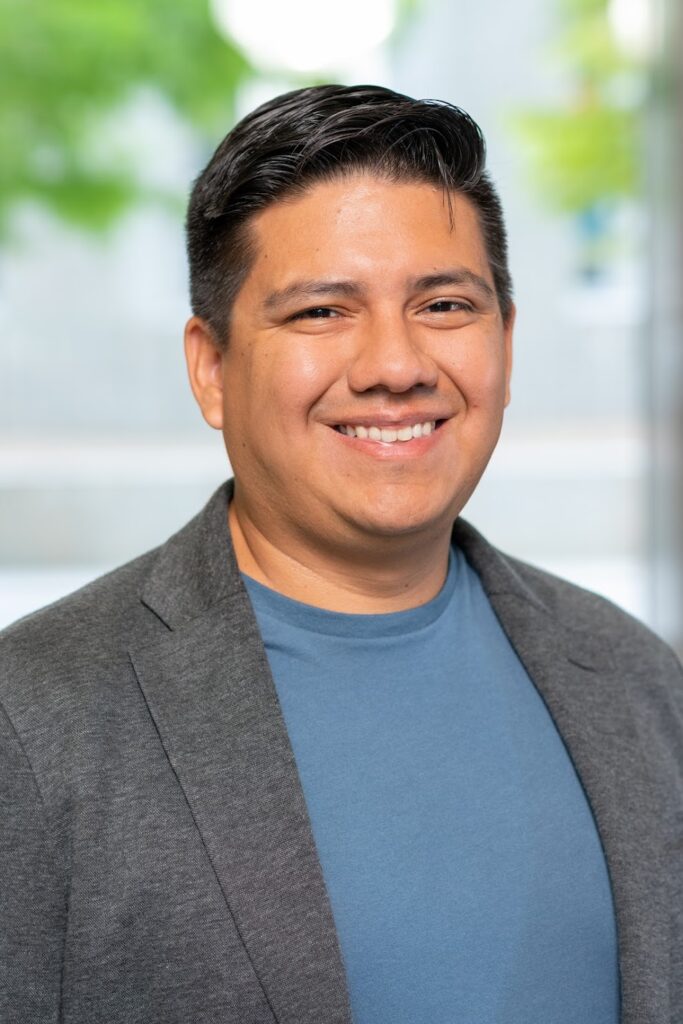
Juan Sequeda, Principal Scientist at data.world. He holds a PhD in Computer Science from The University of Texas at Austin. Juan’s goal is to reliably create knowledge from inscrutable data. His research and industry work has been on designing and building Knowledge Graph for enterprise data integration. Juan has researched and developed technology on semantic data virtualization, graph data modeling, schema mapping and data integration methodologies. He pioneered technology to construct knowledge graphs from relational databases, resulting in W3C standards, research awards, patents, software and his startup Capsenta (acquired by data.world). Juan strives to build bridges between academia and industry as the current co-chair of the LDBC Property Graph Schema Working Group, past member of the LDCB Graph Query Languages task force, standards editor at the World Wide Web Consortium (W3C) and organizing committees of scientific conferences, including being the general chair of The Web Conference 2023. Juan is also the co-host of Catalog and Cocktails, an honest, no-bs, non-salesy podcast about enterprise data.
Resources

Designing and Building Enterprise Knowledge Graphs Synthesis Lectures on Data, Semantics, and Knowledge August 2021, 165 pages, (https://doi.org/10.2200/S01105ED1V01Y202105DSK020) Juan Sequeda, data.world; Ora Lassila, Amazon
Related Posts
Fighting Covid-19 with Graphs. Interview with Alexander Jarasch ODBMS Industry Watch, June 8, 2020
Follow us on Twitter: @odbmsorg
##
“I think that many companies need to understand that their customers are worried about the use of AI and then act accordingly. I believe they should set up ethics advisory boards and then follow the advice or internal teams to advise on what they should do and take that advise.”
–Kay Firth-Butterfield
I have interviewed Kay Firth-Butterfield, Head of Artificial Intelligence and member of the Executive Committee at the World Economic Forum. We talked about Artificial Intelligence (AI) and in particular, we discussed responsible AI, trustworthy AI and AI ethics.
RVZ
Q1. You are the Head of Artificial Intelligence and a member of the Executive Committee at the World Economic Forum. What is your mission at the World Economic Forum?
Kay Firth-Butterfield: We are committed to improving the state of the world.
Q2. Could you summarize for us what are in your opinion the key aspects of the beneficial and challenging technical, economic and social changes arising from the use of AI?
Kay Firth-Butterfield: The potential benefits of AI being used across government, business and society are huge. For example using AI to help find ways of educating the uneducated, giving healthcare to those without it and helping to find solutions to climate change. Both embodied in robots and in our computers it can help keep the elderly in their homes and create adaptive energy plans for air conditioning so that we use less energy and help keep people safe. Apparently some 8800 people died of heat in US last year but only around 450 from hurricanes. Also, it helps with cyber security and corruption. On the other side, we only need to look at the fact that over 190 organisations have created AI principles and the EU is aiming to regulate use of AI and the OHCHR has called for a ban on AI which affects human rights to know that there are serious problems with the way we use the tech, even when we are careful.
Q3. The idea of responsible AI is now mainstream. But why when it comes to operationalizing this in the business, companies are lagging behind?
Kay Firth-Butterfield: I think they are worried about what regulations will come and the R&D which they might lose from entering the market too soon. Also, many companies don’t know enough about the reasons why they need AI. CEOs are not envisaging the future of the company with AI which, if available is often left to a CTO. It is still hard to buy the right AI for you and know whether it is going to work in the way it is intended or leave an organisation with an adverse impact on its brand. Boards often don’t have technologists and so they can help the CEO think through the use of AI for good or ill. Finally, its is hard to find people with the right skills. I think this may be helped by remote working when people don’t have to locate to a country which is reluctant to issue visas.
Q4. What is trustworthy AI?
Kay Firth-Butterfield: The design, development and use of AI tools which do more good for society than they do harm.
Q5. The Forum has developed a board tool kit to help board member on how to operationalize AI ethics. What is it? Do you have any feedback on how useful is it in practice?
Kay Firth-Butterfield: It provides Boards with information which allows they to understand how their role changes when their company uses AI and therefore gives them the tools to develop their governance and other roles to advise on this complex topic. Many Boards have indicated that they have found it useful and it has been downloaded more than 50,000 times.
Q6. Let´s talk about standards for AI. Does it really make sense to standardize an AI system? What is your take on this?
Kay Firth-Butterfield: I have been working with the IEEE on standards for AI since 2015, I am still the Vice-Chair. I think that we need to use all types of governance for AI from norms to regulation depending on risk. Standards provide us with an excellent tool in this regard.
Q7. There are some initiatives for Certification of AI. Who has the authority to define what a certification of AI is about?
Kay Firth-Butterfield: At the moment there are many who are thinking about certification. There is not regulation and no way of being certified to certify! This needs to be done or there will be a proliferation and no-one will be able to understand which is good and which is bad. Governments have a role here, for example Singapore’s work on certifying people to use their Model AI Governance Framework.
Q8. What kind of incentives are necessary in your opinion for helping companies to follow responsible AI practices?
Kay Firth-Butterfield: I think that many companies need to understand that their customers are worried about the use of AI and then act accordingly. I believe they should set up ethics advisory boards and then follow the advice or internal teams to advise on what they should do and take that advise. In our Responsible Use of Technology work we have considered this in detail.
Q9. Do you think that soft government mechanisms would be sufficient to regulate the use of AI or would it be better to have hard government mechanisms?
Kay Firth-Butterfield: both
Q10. Assuming all goes well, what do you think a world with advanced AI would look like?
Kay Firth-Butterfield: I think we have to decide what trade offs of privacy we want to allow for humans to develop harnessing AI. I believe that it should be up to each of us but sadly one person deciding to use surveillance via a doorbell surveills many. I believe that we will work with robots and AI so that we can do our jobs better. Our work on positive futures with AI is designed to help us better answer this question. Report out next month! Meanwhile here is an agenda.
…………………………………………………………

Kay Firth-Butterfield is a lawyer, professor, and author specializing in the intersection of business, policy, artificial intelligence, international relations, and AI ethics.
Since 2017, she has been the Head of Artificial Intelligence and a member of the Executive Committee at the World Economic Forum and is one of the foremost experts in the world on the governance of AI. She is a barrister, former judge and professor, technologist and entrepreneur and vice-Chair of The IEEE Global Initiative for Ethical Considerations in Artificial Intelligence and Autonomous Systems. She was part of the group which met at Asilomar to create the Asilomar AI Ethical Principles, is a member of the Polaris Council for the Government Accountability Office (USA), the Advisory Board for UNESCO International Research Centre on AI and AI4All.
She regularly speaks to international audiences addressing many aspects of the beneficial and challenging technical, economic and social changes arising from the use of AI.
Resources
- Empowering AI Leadership: An Oversight Toolkit for Boards of Directors. World Economic Forum.
- Ethics by Design: An organizational approach to responsible use of technology. White Paper December 2020. World Economic Forum.
- A European approach to artificial intelligence, European Commission.
- The IEEE Global Initiative for Ethical Considerations in Artificial Intelligence and Autonomous Systems
Related Posts
On Digital Transformation and Ethics. Interview with Eberhard Schnebel. ODBMS Industry Watch. November 23, 2020
On the new Tortoise Global AI Index. Interview with Alexandra Mousavizadeh. ODBMS Industry Watch, April 7, 2021
Follow us on Twitter: @odbmsorg
##
I have interviewed Ryan Betts, VP of Engineering at InfluxData. We talked about time series databases, InfluxDB and the InfluxData stack. RVZ
“Time series databases have key architectural design properties that make them very different from other databases. These include time-stamped data storage and compression, data lifecycle management, data summarization, ability to handle large time-series-dependent scans of many records, and time-series-aware queries.“–Ryan Betts
Q1. What is time series data?
Ryan Betts: Time series data consists of measurements or events that are captured and analyzed, often in real time, to operate a service within an SLO, detect anomalies, or visualize changes and trends. Common time series applications include server metrics, application performance monitoring, network monitoring, and sensor data analytics and control loops. Metrics, events, traces and logs are examples of time series data.
Q2. What are the hard database requirements for time series applications?
Ryan Betts: Managing time series data requires high-performance ingest (time series data is often high-velocity, high-volume), real-time analytics for alerting and alarming, and the ability to perform historical analytics against the data that’s been collected. Additionally, many time series applications apply a lifecycle policy to the data collected — perhaps downsampling or aggregating raw data for historical use.
With time series, it’s common to perform analytics queries over a substantial amount of data. Time series queries commonly include columnar scans, grouped and windowed aggregates, and lag calculations. This kind of workload is difficult to optimize in a distributed key value store. InfluxDB uses columnar database techniques to optimize for exactly these use cases, giving sub-second query times over swathes of data and supporting a rich analytics vocabulary.
While time series data is typically structured, it often has dynamic properties that aren’t well-suited to strict schema enforcement. Time series databases often specify the structure of data but allow schema-on-write. Another way of saying this is that time series databases often support arbitrary dimension data to decorate the contents of the fact table. This allows developers to create new instrumentation or collect metrics from new sources without performing frequent schema migrations. Document databases and column-family stores similarly allow flexible schema in their own contexts. The motivation with time series is similar — optimizing for developer productivity.
In addition to high-performance ingest, non-trivial analytics queries, and flexible schema, TSDBs also need to bridge real-time analytics to real-time action. There’s little point doing real-time monitoring if you can’t also automate real-time responses. So time series databases, like other real-time analytics systems, need to provide the analytics function and the ability to tie into real-time operations. That means integrating automated alerting, alarming, and API invocations with the query analytics performed for monitoring.
Q3. How do you manage the massive volumes and countless sources of time-stamped data produced by sensors, applications and infrastructures?
Ryan Betts: The InfluxData stack is optimized for both regular (metrics often gathered from software or hardware sensors) and irregular time series data (events driven either by users or external events), which is a significant differentiator from other solutions like Graphite, RRD, OpenTSDB, or Prometheus. Many services and time series databases support only the regular time series metrics use case.
InfluxDB lets users collect from multiple and diverse sources, store, query, process and visualize raw high-precision data in addition to the aggregated and downsampled data. This makes InfluxDB a viable choice for applications in science and sensors that require storing raw data.
At the storage level, InfluxDB organizes data into a columnar format and applies various compression algorithms, typically reducing storage to a fraction of the raw uncompressed size. Time series applications are “append-mostly”. The majority of arriving data is appended. Late arriving data and deletes occur with some frequency — but primarily writes result in appending to the fact table. The database uses a log structured merge tree architecture to meet these requirements. Deletes are recorded first as tombstones and are later removed through LSM compaction.
Q4. Can you give us some time series examples?
Ryan Betts: Time series data, also referred to as time-stamped data, is a sequence of data points indexed in time order. Time-stamped is data collected at different points in time.
These data points typically consist of successive measurements made from the same source over a time interval and are used to track change over time.
Weather records, step trackers, heart rate monitors, all are time series data. If you look at the stock exchange, a time series tracks the movement of data points, such as a security’s price over a specified period of time with data points recorded at regular intervals.
InfluxDB has a line protocol for sending time series data which takes the following form:
<measurement name>,<tag set> <field set> <timestamp>
The measurement name is a string, the tag set is a collection of key/value pairs where all values are strings, and the field set is a collection of key/value pairs where the values can be int64, float64, bool, or string. The measurement name and tag sets are kept in an inverted index which makes lookups for specific series very fast.
For example, if we have CPU metrics:
cpu,host=serverA,region=uswest idle=23,user=42,system=12 1549063516
Timestamps in InfluxDB can be by second, millisecond, microsecond, or nanosecond precision. The micro and nanosecond scales make InfluxDB a good choice for use cases in finance and scientific computing where other solutions would be excluded. Compression is variable depending on the level of precision the user needs.
Q5. The fact that time series data is ordered makes it unique in the data space because it often displays serial dependence. What does it mean in practice?
Ryan Betts: Serial dependence occurs when the value of a datapoint at one time is statistically dependent on another datapoint at another time.
Though there are no events that exist outside of time, there are events where time isn’t relevant. Time series data isn’t simply about things that happen in chronological order — it’s about events whose value increases when you add time as an axis. Time series data sometimes exists at high levels of granularity, as frequently as microseconds or even nanoseconds. With time series data, change over time is everything.
Q6. How is time series data understood and used?
Ryan Betts: Time series data is gathered, stored, visualized and analyzed for various purposes across various domains:
- In data mining, pattern recognition and machine learning, time series analysis is used for clustering, classification, query by content, anomaly detection and forecasting.
- In signal processing, control engineering and communication engineering, time series data is used for signal detection and estimation.
- In statistics, econometrics, quantitative finance, seismology, meteorology, and geophysics, time series analysis is used for forecasting.
Time series data can be visualized in different types of charts to facilitate insight extraction, trend analysis, and anomaly detection. Time series data is used in time series analysis (historical or real-time) and time series forecasting to detect and predict patterns — essentially looking at change over time.
Q7. You also handle two other kinds of data, namely cross-section and panel data. What are these? How do you handle them?
Cross-sectional data is a collection of observations (behavior) for multiple entities at a single point in time. For example: Max Temperature, Humidity and Wind (all three behaviors) in New York City, SFO, Boston, Chicago (multiple entities) on 1/1/2015 (single instance).
Panel data is usually called cross-sectional time series data, as it is a combination of both time series data and cross-sectional data (i.e., collection of observations for multiple subjects at multiple instances).
This collection of data can be combined in a single series, or you can use Flux lang to combine and review this data to gather insights.
Q8. There are several time series databases available in the market. What makes InfluxDB time series database unique?
Ryan Betts: When doing a comparison, the entire InfluxDB Platform should be taken into account. There are multiple types of databases that get brought up for comparison. Mostly, these are distributed databases like Cassandra or more time-series-focused databases like Graphite or RRD. When comparing InfluxDB with Cassandra or HBase, there are some stark differences. First, those databases require a significant investment in developer time and code to recreate the functionality provided out of the box by InfluxDB. Finally, they’ll have to create an API to write and query their new service.
Developers using Cassandra or HBase need to write tools for data collection, introduce a real-time processing system and write code for monitoring and alerting. Finally, they’ll need to write a visualization engine to display the time series data to the user. While some of these tasks are handled with other time series databases, there are a few key differences between the other solutions and InfluxDB. First, other time series solutions like Graphite or OpenTSDB are designed with only regular time series data in mind and don’t have the ability to store raw high-precision data and downsample it on the fly.
While with other time series databases, the developer must summarize their data before they put it into the database, InfluxDB lets the developer seamlessly transition from raw time series data into summarizations.
InfluxDB also has key advantages for developers over Amazon Timestream. Among them:
- InfluxData is first and foremost an open source company. It is committed to sharing ideas and information openly, collaborating on solutions and providing full transparency to drive innovation.
- Hybrid cloud and on-premises support. Every business has specific functionalities, and a hybrid cloud system offers the flexibility to choose services that best fit their needs, whether to support GDPR regulatory requirements or teams that are spread across multiple providers.
Q9. What distinguishes the time series workload?
Ryan Betts: Time series databases have key architectural design properties that make them very different from other databases. These include time-stamped data storage and compression, data lifecycle management, data summarization, ability to handle large time-series-dependent scans of many records, and time-series-aware queries.
For example: With a time series database, it is common to request a summary of data over a large time period. This requires going over a range of data points to perform some computation like a percentile increase this month of a metric over the same period in the last six months, summarized by month. This kind of workload is very difficult to optimize for with a distributed key value store. TSDB’s are optimized for exactly this use case giving millisecond- level query times over months of data.
Q10. Let’s talk about integrations. Software services don’t work alone. Suppose an application relies on Amazon Web Services, or monitors Kubernetes with Grafana or deploys applications through Docker, how easy is it to integrate them with InfluxDB?
Ryan Betts: InfluxData provides tools and services that help you integrate your favorite systems across the spectrum of IT offerings, from applications to services, databases to containers. We currently offer 200+ Telegraf plugins to allow these seamless integrations. Developers using the InfluxDB platform build their applications with less effort, less code, and less configuration with the use of a set of powerful APIs and tools. InfluxDB client libraries are language-specific tools that integrate with the InfluxDB API and can be used to write data into InfluxDB as well as query the stored data.
………………………………………………..

Ryan Betts is VP of Engineering at InfluxData. Ryan has been building high performance infrastructure software for over twenty years. Prior to InfluxData, Ryan was the second employee and CTO at VoltDB. Before VoltDB, he spent time building SOA security and core networking products. Ryan holds a B.S. in Mathematics from Worcester Polytechnic Institute and an MBA from Babson College.
Resources
influxdata/influxdb: Scalable datastore for metrics – GitHub
Introduction to Time Series Databases | Getting Started [1 of 7] YouTube
Related Posts
COVID-19 Tracking Using Telegraf and InfluxDB Dashboards
On Big Data Benchmarking. Q&A with Richard Stevens
The 2021 AI Index report (HAI Stanford University)
Follow us on Twitter: @odbmsorg
##
“The most dangerous pitfall is when you solve the wrong problem.” –Joyce Weiner
I have interviewed Joyce Weiner, Principal AI Engineer at Intel Corporation. She recently wrote a book on Why AI/Data Science Projects Fail.
RVZ
Q1. In your book you start by saying that 87% of Artificial Intelligence/Big Data projects don’t make it into production, meaning that most projects are never deployed. Is this still actual?
Joyce Weiner: I can only provide the anecdotal evidence that it is still a topic of conversation at conferences and an area of concern. A quick search doesn’t provide me with any updated statistics. The most recent data point appears to be the Venture Beat reference (VB Staff, 2019). Back in 2019, Gartner predicted that “Through 2022, only 20% of analytic insights will deliver business outcomes.” (White, 2019)
Q2. What are the common pitfalls?
Joyce Weiner: I specifically address the common pitfalls that are in the control of the people working on the project. Of course, there can be other external factors that will impact a project’s success. But just focusing on what you can control and change:
- The scope of the project is too big
- The project scope increased in size as the project progressed (scope creep)
- The model couldn’t be explained
- The model was too complex
- The project solved the wrong problem
Q3. You mention five pitfalls, which of the five are most frequent?, and which one are the most dangerous for a project?
Joyce Weiner: Of the five pitfalls, scope creep has been the one I have seen the most in my experience. It’s an easy trap to fall into, you want to build the best solution and there is a tendency to add features when they come to mind without assessing the amount of value they add, or if it makes sense to add them right now. The most dangerous pitfall is when you solve the wrong problem. In that case, not only have you spent time and effort on a solution, once you have realized that you solved the wrong problem, you now need to go and redo the project to target the correct problem. Clearly, that can be demoralizing for the team working on the project, not to mention the potential business impact from the delay in delivering a solution.
Q4. You suggest five methods to avoid such pitfalls. What are they?
Joyce Weiner: The five methods I discuss in the book to avoid the pitfalls mentioned previously are:
- Ask questions – this addresses the project scope as well as providing information to decide on the amount of explainability required, and most importantly, ensures you are solving the correct problem.
- Get alignment – working with the project stakeholders and end users, starting as early as the project definition and continuing throughout the project, addresses problems with project scope and makes sure you are on track to solve the correct problem
- Keep it simple – this addresses model explainability and model complexity
- Leverage explainability – obviously directly related to model explainability, and addresses the pitfall of solving the wrong problem
- Have the conversation – continually discussing the project, expected deliverables, and sharing mock-ups and prototypes with your end users as you build the project addresses all 5 of the project pitfalls.
Q5. How do you apply and measure effectivnesss of these methods in practice?
Joyce Weiner: Well, the most immediate measurement is if you were able to deploy a solution into production. As a project progresses, you can measure things that will help you stay on track. For example, having a project charter to document and communicate your plans becomes a reference point as you build a project so that you recognize scope creep. A project charter is also useful when having conversations with project stakeholders to document alignment on deliverables.
Q6. Throughout your book you use the term “data science projects” as an all-encompassing term that includes Artificial Intelligence (AI) and Big Data projects. Don’t you think that this is a limitation to your approach? Big Data projects might have different requirements and challenges than AI projects?
Joyce Weiner: Well, that is true Big Data projects do have additional challenges, especially around the data pipeline. The five pitfalls still apply, and those are the biggest challenges to getting a project into deployment based on my experience.
Q7. In your book you recommend as part of the project charter to document the expected return on investment for the project. You write that assessing the business value for your project will help get resources and funding. What metrics do you suggest for this?
Joyce Weiner: I propose several metrics in my book, which depend on the type of project you are delivering. For example, a common data science project is performing data analysis. Deliverables for this type of project are root cause determination, problem solving support, and problem identification. Metrics are productivity, which can be measured as time saved, time to decision which is how long it takes to gather the information needed to make a decision, decision quality, and risk reduction due to improved information or consistency in the information used to make decisions.
Q8. You also write that in acquiring data, there are two cases. One, when the data are available already either in internal systems or from external sources, and two, when you don’t have the data. How do you ensure the quality (and for example the absence of Bias) of the existing data?
Joyce Weiner: The easiest way to ensure you have high quality data is to automate data collection as much as possible. If you rely on people to provide information, make it easy for them to enter the data. I have found that if you require a lot of fields for data entry, people tend to not fill things in, or they don’t fill things in completely. If you can collect the data from a source other than a human, say ingesting a log file from a program, your data quality is much higher. Checking for data quality by examining the data set before beginning on any model building is an important step. You can see if there are a lot of empty fields or gaps, or one-word responses in free text fields – things that call the quality of the data into question. You also get a sense of how much data cleaning you’ll need to do.
Bias is something that you need to be aware of, for example, if your data set is made solely of failing samples, you have no information on what makes something good or bad. You can only examine the bad. Building a model from those data that “predicts” good samples would be wrong. I’ve found that thinking through the purpose of the data and doing it as early as possible in the process is key. Although it’s tempting to say, “given these data, what can I do?” it’s better to start from a problem statement and then ensure you are collecting the proper data related to the problem to avoid having a biased data set.
Q9. What do you do if you do not have any data?
Joyce Weiner: Well, it makes it very difficult to do a data science project without any data. The first thing to do is to identify what data you would want if you could have them. Then, develop a plan for collecting those data. That might be building a survey or that might mean adding sensors or other instruments to collect data.
Q10. How do you know when an AI/Big Data Project is ready for deployment?
Joyce Weiner: In my experience a project is ready for deployment when you have aligned with the end user and have completed all the items needed to deliver the solution they want. This includes things like a maintenance plan, metrics to monitor the solution, and documentation of the solution.
Q11. Can you predict if a project will fail after deployment?
Joyce Weiner: If a project doesn’t start well, meaning if you aren’t thinking about deployment as you build the solution, it doesn’t bode well for the project overall. Without a deployment plan, and without planning for things like maintainability as you build the project, then it is likely the project will fail after deployment. And by this I include a dashboard which doesn’t get used, or a model that stops working and can’t be fixed by the current team.
Q12. What measures do you suggest to monitor a BigData/AI project after it is deployed?
Joyce Weiner: The simplest measure is usage. If the solution is a report, are users accessing it? If it’s a model, then also adding predicted values versus actual measurements. In the book, I share a tool called a SIPOC or supplier-input-process-output-customer which helps identify the metrics the customer cares about for a project. Some examples are timeliness, quality, and support level agreements.
Q13. In your book you did not address the societal and ethical implications of using AI. Why?
Joyce Weiner: I didn’t address the societal and ethical implications of AI for two reasons. One, it isn’t my area of expertise. Second, it is such a big topic that it warrants its own book.
……………………………………
Joyce Weiner is a Principal AI Engineer at Intel Corporation. Her area of technical expertise is data science and using data to drive efficiency. Joyce is a black belt in Lean Six Sigma. She has a BS in Physics from Rensselaer Polytechnic Institute, and an MS in Optical Sciences from the University of Arizona. She lives with her husband outside Phoenix, Arizona.
References
VB Staff. (2019, July 19). Why do 87% of data science projects never make it into production? Retrieved from VentureBeat: https://venturebeat.com/2019/07/19/why-do-87-of-data-science-projects-never-make-it-into-production/
White, A. (2019, Jan 3). Our Top Data and Analytics Predicts for 2019. Retrieved from Gartner: https://blogs.gartner.com/andrew_white/2019/01/03/our-top-data-and-analytics-predicts-for-2019/